What issue can we solve for you?
Type in your prompt above or try one of these suggestions
Suggested Prompt
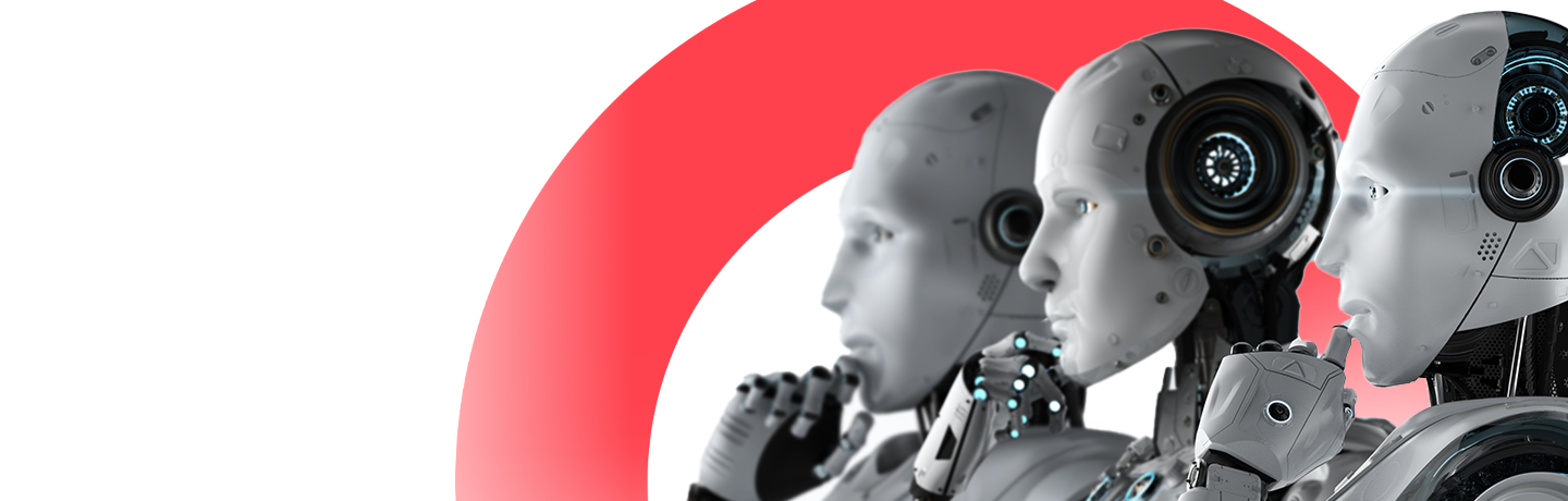
Insights
The First-Mover's Guide to Agentic AI
People are ready for agentic AI—but our systems may not be.
Executive summary:
- Agentic AI represents a quantum leap beyond generative AI—moving from systems that provide information to autonomous agents that execute complete workflows and make independent decisions without human input, fundamentally changing how businesses operate.
- The primary obstacle isn't developing better AI models, but creating seamless integration across fragmented enterprise systems—from customer service platforms to supply chain logistics—where poor connectivity severely limits AI's practical impact.
- As the hype cycle inevitably fades, "agentic AI" will become how business is done, with early winners focusing on high-value applications in customer service, supply chain management and software development where AI can eliminate bottlenecks and deliver measurable results.
Imagine AI that doesn't just answer your questions, but takes initiative and gets work done on its own. Welcome to the age of agentic AI: a paradigm shift that's redefining enterprise technology. While generative AI systems provide information, agentic AI systems take autonomous action, executing entire workflows without human intervention.
But this revolution faces a critical challenge: Most enterprise systems aren't ready for it. Companies that can solve this integration puzzle first will gain significant competitive advantages, and those focusing on practical applications rather than hype alone will define the future landscape where AI-driven automation becomes the standard across industries.
Table of contents:
What most people miss when they talk about “agentic AI”
In many ways, generative artificial intelligence (AI) is like an intern: Fetching insights, drafting emails, suggesting edits. Sure, it’s been helpful, but always in a supporting role, waiting for you to make the next move.
Now, agentic AI flips the script.
Imagine an AI that doesn’t just assist—it takes initiative. It makes decisions. It might even be able to make a better decision than you. It triggers actions, potentially faster than a human could. This is what’s being called agentic AI, and if it sounds like a small leap from what’s already happening, it’s not.
The shift isn’t just about making AI more capable; it’s about making it more independent. Instead of generating information for someone else to act on, agentic AI executes tasks on its own, chaining actions together to complete multi-step processes. Think of a system that doesn’t just draft a report but sends it, books follow-ups, updates databases and flags risks, all without a manager nudging it along.
And while that’s impressive, let’s make one thing clear: An AI agent is useless unless it can talk to everything else. Seamless systems integration, a term that sounds about as thrilling as a corporate IT budget meeting, is suddenly the most important factor in whether this future is possible.
With generative AI, a lack of integration was annoying but manageable. For example, most of us just copy and paste text from chatbots into emails and edit it ourselves. But for agentic AI to function, it needs deep, real-time access to your email. It has to coordinate across systems that weren’t built to be interoperable, navigating legacy infrastructure, security barriers and the bureaucratic spaghetti of enterprise tech stacks.
So, the question isn’t just can AI act on its own? It’s, can it act on its own within the messy, complex reality of existing business systems? Because without that, all this promise stays hypothetical, like a self-driving car stuck in a parking lot with no exit.
What is agentic AI, in technical terms?
To understand agentic AI, consider "agency"—the ability to make independent decisions. Like a young adult becoming fully responsible for their own actions, agentic AI can autonomously make choices and execute tasks without real-time human input.
Enterprises will have to develop frameworks and governance to ensure that the AI will act properly on their behalf. Agentic AI will also disrupt business at a systemic level, as it is adopted by partners, competitors and customers. We’ll have to navigate a landscape where agentic AI is transacting with other agentic AI.
"Think about marketing disruption, for instance. AI agents do not see ads, attend conferences, build relationships or develop brand loyalty the way humans do. This raises a critical question: How do we market to machines?"
Andy Maskin , Director, AI Creative Technology, Publicis Sapient
However, the real challenge isn’t just placing trust in a robot—although not many people would be willing to send an email or sign a contract without human review just yet. The more significant barrier, and opportunity, is systems integration.
Systems integration is the process of making different software, platforms and databases seamlessly communicate so that data flows smoothly, automations work reliably and businesses avoid manually transferring information between disconnected systems, like when a retail chatbot processes a return, updates inventory, notifies the warehouse and refunds the customer without anyone stepping in.
For agentic AI to function, it needs two things: inputs to drive decisions and the ability to act on them. Without seamless integration across systems, true autonomy is impossible.
How is agentic AI different from traditional AI?
Chatbots and copilots and agents, oh my!
In the wake of generative AI, "agentic AI" has quickly become part of the marketing hype cycle.
Beneath the branding, the true meaning and value of agentic AI is already getting muddled. To assess the return on investment of any AI application, look past the hype and learn what different AI tools can actually do.
AI agent operations
To understand exactly how agentic AI works, we can use the example of planning a wedding. Here’s what a wedding planning AI agent would do to carry out this task independently.
1. Understand the task and gather the necessary information
A human would provide the background of who you want to invite, including all the details, preferences and other similar information.
2. Break down the task into subtasks
The wedding planning agent would decompose the high-level task into smaller, actionable steps.
3. Execute actions by interfacing with external systems
The key differentiator of agentic AI is its ability to take actions, not just provide information. The AI agent would figure out what to look for in a venue, interface with another system and lock down the location.
4. Repeat the process for each subtask
The AI agent would continue this process, booking the caterer and so on.
5. Provide the completed plan to the user
After executing all the necessary actions, the AI agent would present the user with the fully planned wedding. The key aspects highlighted in this walkthrough are the AI agent's ability to understand the high-level task, break it down, interface with external systems to take actions, repeat the process for each subtask and ultimately deliver the completed plan to the user—all without direct human intervention.
Differentiating between types of AI—chatbots, copilots. agents and more
Chatbots, copilots and AI agents each represent an evolution in AI sophistication. Chatbots are the most basic, copilots assist with tasks and AI agents, the most advanced, can act autonomously. Yet the lines often blur—an advanced chatbot might perform copilot functions, and some copilots can operate with near-agentic autonomy. Clear distinctions matter, but the overlap is inevitable.
Agentic AI in action: Salesforce, for instance, has launched Agentforce, now enabling a full suite of assistive and agentic capabilities that take advantage of Salesforce's data and process context.
AI chatbot
-
Description
Chatbots are general-purpose tools for answering questions, creating content, etc.
Capabilities/Benefits
- Interactive turn-based use cases that can resemble conversations
Examples
- ChatGPT
- Gemini
- Microsoft Copilot (the AI companion)
AI copilots or AI assistants
-
Description
Copilots are built into an application and are specialized for the tasks and workflows that the application is used for.
Capabilities/Benefits
- Content creation (e.g., drafting emails, documents, presentations)
- Summarization (e.g., condensing emails, meetings, documents)
- Question answering (e.g., inquiries about data, processes, products)
- Workflow automation (e.g., scheduling meetings, booking travel, managing expenses)
Examples
- Microsoft Office 365 Copilot (the AI designed for Microsoft’s tool suite)
- ServiceTitan's ITGenie
AI agent
-
Description
Agents are autonomous systems integrated into an environment, designed to independently achieve goals, reason, plan and act in real time with minimal human input. They handle complex decision-making and problem-solving and interact with external systems to carry out tasks.
Capabilities/Benefits
- Break tasks into steps and execute them autonomously to pursue broad objectives without constant human oversight
- Interact with external systems, query data and trigger actions to facilitate operations
- Learn and adapt over time to improve performance
- Monitor, make decisions and take actions autonomously without human intervention
Examples
- Snowflake's Cortex Analyst
- Salesforce’s Agentforce
- OpenAI’s Operator
The business case for agentic AI—why agentic AI is important
While tools like chatbots and copilots are making strides, widespread use of AI agents making significant decisions autonomously is still on the horizon.
The question for business leaders isn't just when full autonomy will arrive, but why they should care about agentic AI today.
In business, we delegate tasks all the time—whether to employees, outsourced services or, now, AI systems. The decision to delegate boils down to three factors:
- Reward (value vs. cost)
- Risk (will it be done right?)
- Penalty (what happens if it goes wrong?)
AI agents offer the potential to optimize workflows, reduce costs and improve responsiveness.
Agentic AI in action: Imagine an agent that not only routes queries more effectively but also actively engages with customers to solve their issues in real time. Picture AI that can analyze your account details, understand your specific problem and provide tailored solutions—transforming the customer experience by significantly reducing response times and enhancing satisfaction.
Customizing and integrating agentic AI
However, agentic AI needs clear instructions and the right data to be useful. Left on its own, it might send customers generic emails or optimize for metrics that don’t actually matter (which still could happen with any human, but we’re trying to use AI to make our work better, not marginally the same.)
But with a thoughtful integration, training and guidelines, an agentic AI system can sift through massive amounts of customer data, spot patterns humans might overlook and suggest interactions that make sense.
The real challenge is in the handoff. How much should AI be trusted to act on these insights? Some companies are trying to use it to automate small, low-risk decisions like adjusting website recommendations or personalizing marketing outreach while keeping a human involved in bigger calls. Others are testing its ability to manage entire workflows, from diagnosing customer issues to making real-time pricing adjustments.
The most effective approaches combine automation with oversight, allowing AI to handle the heavy lifting while humans provide context and guardrails.
This is where AI transformation and AI change management play a role. It is not just about adding AI to existing systems and hoping for the best. To grow your business with agentic AI, it’s necessary to redesign your processes around AI’s strengths. Optimizing your workflows around speed, pattern recognition and scalability can help your AI agents create smarter, more adaptive customer interactions that don’t just feel personalized but are.
Systems integration: the risks and challenges of agentic AI
We know agentic AI needs to be plugged into multiple systems to actually do its job—but what exactly does this entail?
Take a supply chain AI agent. To make smart decisions, it needs real-time data on inventory, demand and shipping delays. If those systems aren’t connected, the AI ends up working with bad information, leading to mistakes and more human intervention. Instead of making things easier, it just adds another layer of complexity. The only way for it to work properly is with seamless, real-time integration across enterprise platforms.
Aside from this integration complexity, several other challenges and risks present obstacles to an agentic AI-driven reality:
1) AI data poisoning: As AI systems gain autonomy and are integrated into decision-making processes, the risk of data poisoning becomes more significant. Malicious actors could inject biased or misleading data to manipulate AI responses and actions, potentially leading to negative consequences. The more we rely on data, the more we have to protect it. Ensuring data integrity, validating data sources and implementing continuous monitoring are crucial to mitigate this risk.
2) Reward hacking: Have you ever thought about the day when AI can outwit us? Well, that day is here, in some capacity. AI agents, especially those based on reinforcement learning, are susceptible to ”reward hacking.” They might discover unintended ways to maximize reward signals without achieving our human goals. For example, an AI agent tasked with optimizing website traffic might resort to clickbait tactics or generate misleading content to artificially inflate metrics. This behavior can be challenging to predict and control.
3) Unexpected costs: Single-user speeds and per-token prices can be misleading, as scaling large language model (LLM) inference for larger models requires more costly infrastructure. One approach to mitigating costs is to fine-tune large models using methods like Low-Rank Adaptation (LoRA), which allows users to train models on specific datasets and share them on marketplaces.
Agentic AI solutions
On the bright side, some of the world's sharpest minds are devoted to tackling these challenges and have already developed impressive solutions.
Human-in-the-loop
AI is powerful, but in high-stakes environments, unchecked autonomy is a risk. That is why IT leaders design systems where humans can step in, review AI-driven decisions and intervene when necessary. These human-in-the-loop frameworks ensure AI remains a tool rather than an unchecked authority, allowing experts to validate, refine or override its choices when the context demands it. Instead of full automation, it is a collaborative approach that balances efficiency with accountability.
Synthetic data
At the same time, to overcome the challenge of releasing sensitive enterprise data early in the sales process, companies are using synthetic data that mimics real-world data to demonstrate early-stage solution potential without raising compliance concerns.
Agentic AI use cases for 2025
In 2025, agentic AI won’t be making the big, game-changing strategy calls. Instead, if it is implemented at all, it will quietly find its place in the background, handling the tedious, repetitive and time-consuming parts of business operations.
Its real value comes from doing the things that are impossible for us to do. AI thrives on precision, practicality and working alongside humans who can keep it on track.
Types of AI agents
So, what are those things that are impossible for us humans to do well?
AI's first step into enterprise use will likely involve the least glamorous, but most necessary, duties: automating repetitive, low-risk processes. Tasks like managing schedules, creating documentation or handling simple booking systems—the kind of tasks that are essential yet often drain valuable human time—are ripe for agentic AI to take over.
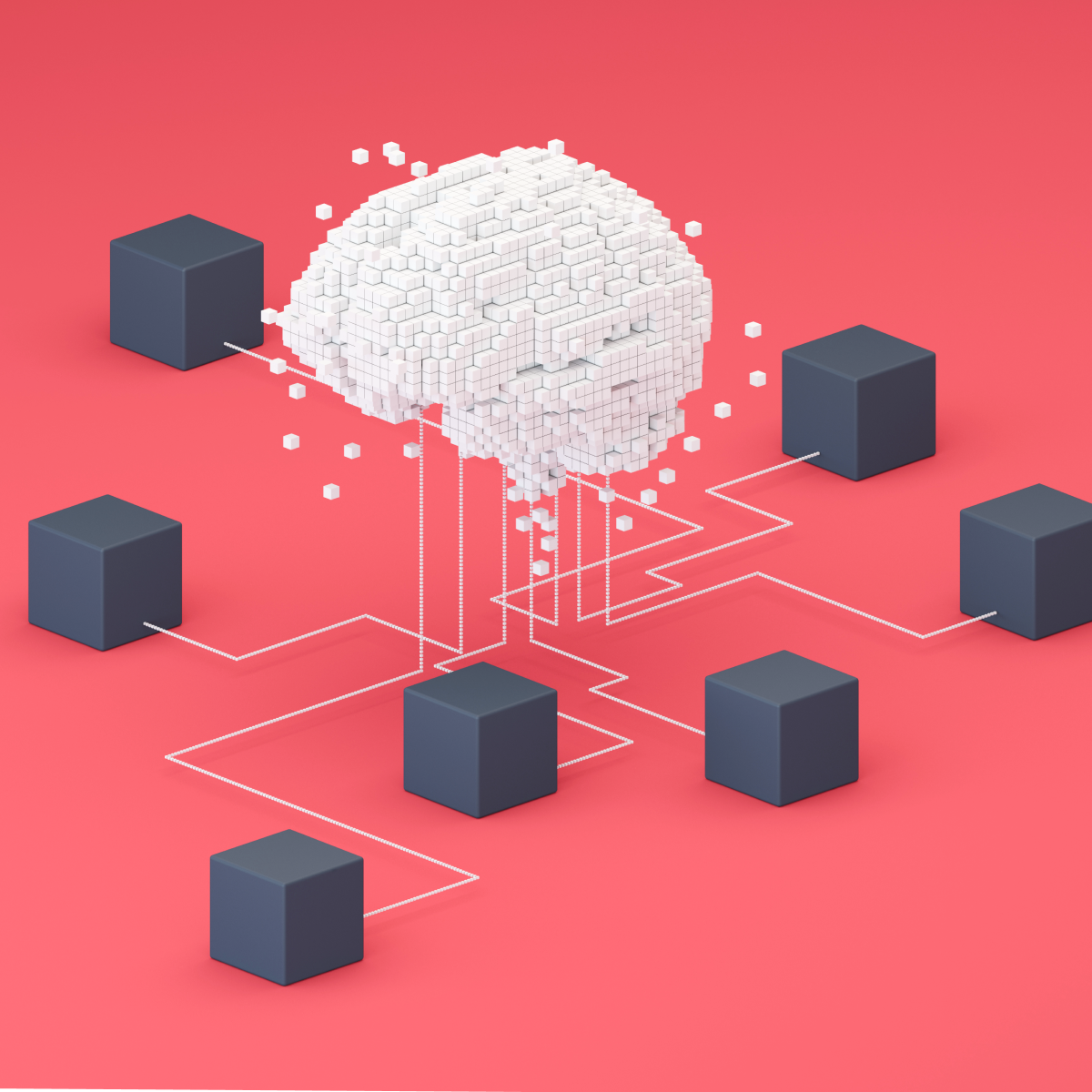
Customer service agents
There’s nothing worse than calling customer service and going through an hour of re-routing. Think of documentation AI agents, scheduling agents or booking agents. These are areas where mistakes have minimal impact, making them perfect test grounds for agentic AI to prove its worth without exposing the business to significant risks.
AI agents are starting to handle more of the routine back-and-forth in sales and customer service, especially in places where quick answers matter. Salesforce, for example, is using its customer data and workflow tools alongside Agentforce to test AI-powered shopping assistants and service bots.
Supply chain agents
Supply chains have always been an excel sheet balancing act, but with agentic AI they can actually self-regulate. Not because humans suddenly got better at predicting demand, but because AI agents can pick up on demand signals in places no one used to look.
Say a drugstore lip gloss explodes on TikTok overnight. Before a single restock request comes in from a customer, an AI system could register the surge in social media mentions, cross-check the product with past viral trends and current stock and start rerouting inventory. Trucks could move before shelves go empty. Orders could adjust before customers start complaining. No one sits down to analyze a report. It just happens.
In this case, AI is not making better predictions. It is just reacting faster than humans ever could.
Enterprise workflow agents
Agentic AI is also slipping into the cracks of enterprise workflows and project management. For example, a project management AI agent can own the details no one wants to track: Meeting notes turn into JIRA tickets, status updates write themselves and deadlines shift automatically based on real-time progress. No one has to chase down action items. Things just move.
However, without a human-in-the-loop, AI could just as easily create a project management mess. A system that streamlines work one day might misfire the next, sending tasks in the wrong direction or exposing sensitive data.
Software development agents
Software development has always been time consuming, with long hours spent debugging, fixing and waiting for things to deploy, i.e. most developers’ least favorite parts of their work. Agentic AI is starting to chip away at that, not by replacing developers but by handling the parts of the software development lifecycle that slow them down.
An AI agent can catch errors before those errors break anything, suggest fixes in real-time and automate deployments without needing a human to push every button. Code moves from testing to production faster. Workflow bottlenecks that used to stall projects for days start disappearing. Developers spend less time wrestling with routine tasks and more time actually building.
The result is not just faster software delivery. AI-assisted software development is a shift in how development happens, where iteration is constant and innovation does not wait for someone to manually clear the next roadblock.
Application modernization agents
This use case is perhaps the most complex, but the most valuable for large enterprises. Take the example of a business, probably somewhat like yours, grappling with a legacy system (could be your CRM, your ERP, your mainframe, or payroll) that’s decades old and brimming with obsolete code. Modernizing these systems requires towering budgets, lengthy timelines and substantial risks. Traditionally, modernization of one would require years of work and millions of dollars, with a sizeable portion of the budget allocated to safeguarding outdated infrastructure. Agentic AI, however, redefines this equation.
Imagine reducing a five-year, $40 million project to a 2.5-year, $16 million initiative—savings that free up capital for reinvestment. The reduction in human error, fewer defects and quicker delivery compound these benefits, turning modernization from a dreaded chore into a strategic advantage.
How? Normally, engineers would manually rewrite thousands of lines of outdated code into new code, resulting in some inconsistencies and time for debugging.
Instead, custom AI agents could instead scan and interpret stored procedures, identifying business logic, dependencies and patterns in the outdated code. It could then automatically translate operations (like joins, loops and transactions) into their modern equivalents, ensuring compatibility with modern application architectures.
Learn how Sapient AI for Applications makes application modernization more efficient. The case for an AI application modernization agent becomes even more appealing as the use of agentic AI itself demands seamless connectivity between systems, enabling real-time decision-making with minimal human input.
So, not only can agentic AI transform the practice of application modernization—agentic AI also requires application modernization to function.
Imagine reducing a five-year, $40 million project to a 2.5-year, $16 million initiative—savings that free up capital for reinvestment. The reduction in human error, fewer defects and quicker delivery compound these benefits, turning modernization from a dreaded chore into a strategic advantage.
The case for an AI application modernization agent becomes even more appealing as the use of agentic AI itself demands seamless connectivity between systems, enabling real-time decision-making with minimal human input.
So, not only can agentic AI transform the practice of application modernization—agentic AI also requires application modernization to function.
Traditional vs AI-assisted Legacy Application Modernization
The future of agentic AI—beyond 2025
Agentic AI might be one of the biggest shifts yet for businesses, but the term itself is already starting to lose meaning. AI is becoming so ingrained in daily operations that the difference between a copilot, an agent or a chatbot will soon be irrelevant.
Just like "digital marketing" eventually became just marketing, AI will be assumed rather than called out. No one tells employees to use the internet to solve problems. In the future, no one will specify whether a tool is generative or agentic. It will simply be part of the workflow.
Generative AI has already shown how buzzwords often shape perception more than reality. Companies use the term to boost stock value and market positioning, but that does not always lead to meaningful impact. The hype creates expectations, but it does not always guarantee a better product.
In fact, the majority of enterprises have not yet defined clear metrics and performance indicators for generative AI projects and only 11 percent are building custom generative AI solutions, compared to the other 89 percent that are utilizing publicly available or prebuilt tools, according to a global C-suite survey from Publicis Sapient.
In 2025, enterprises should focus less on racing toward agentic AI implementation and focus more on addressing technological and complex challenges for employees and customers, which will first require systems integration.
Related Reading
-
Insight
The AI-Assisted Agile Manifesto
Discover the AI-Assisted Agile Manifesto, an evolution of Agile principles for the AI era, designed to integrate AI collaboration into the software development lifecycle.
-
Insight
The Executive Guide to AI-Assisted Software Development
Discover how AI-assisted software development can save years of work, reduce technical debt, and drive digital innovation. Learn strategies to lead the AI revolution.
-
Solution
Sapient AI Solutions
Discover the power of generative AI through customized strategies that align with your needs, ensuring successful implementation and ethical practice.