What issue can we solve for you?
Type in your prompt above or try one of these suggestions
Suggested Prompt
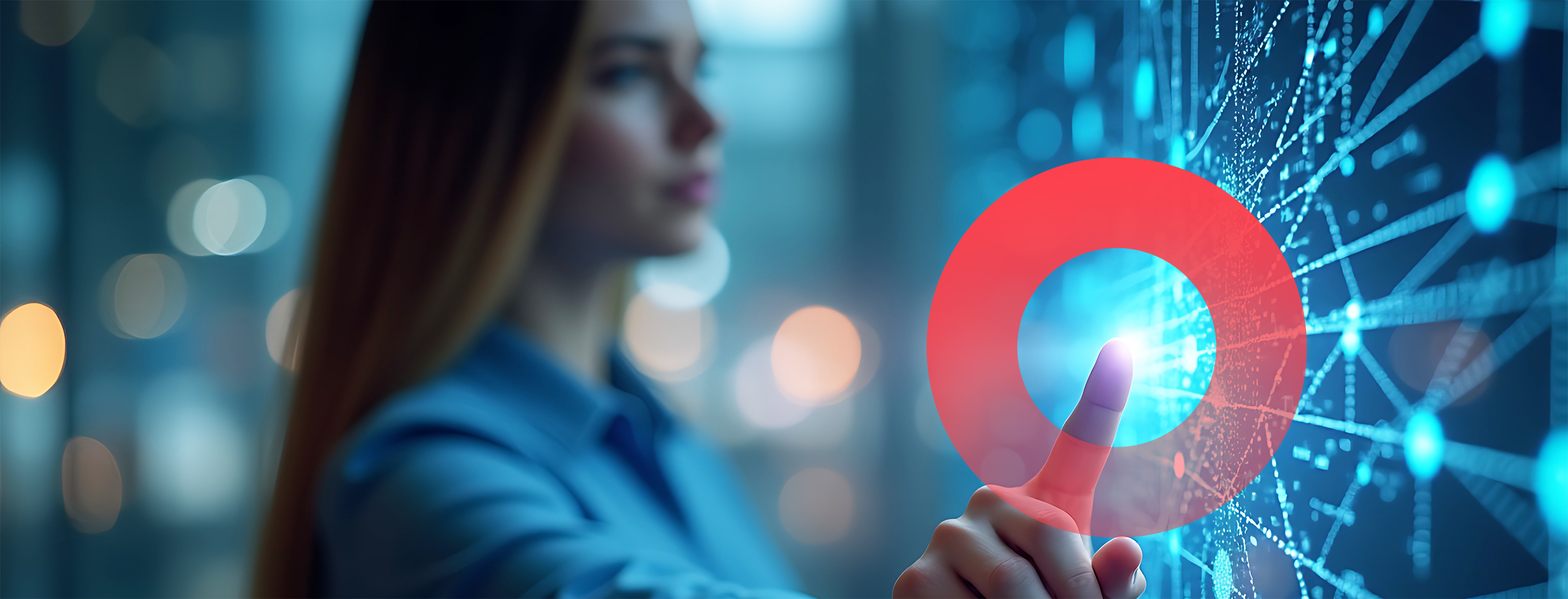
Insight
Getting Started with AI: A Grounded Approach
Getting Started with AI: A Grounded Approach
How to start using AI in practical, incremental ways within the Salesforce ecosystem.
AI has been around for decades but largely remained under the radar for those outside the tech and science industries. The appearance of Generative AI (Gen AI) within the last few years has brought AI into the mainstream, creating an explosive hype cycle.
While the hype has created interest, it hasn’t necessarily sparked adoption. A recent CIO Dive article states, “Organizations are bullish on what AI can do for their operations long-term, but most aren’t ready for widescale adoption just yet.” In fact, “More than 3 in 5 organizations have significant gaps in AI readiness when it comes to infrastructure and data ecosystems.”
AI is not new to Salesforce
Salesforce has been innovating with AI in the CRM industry for several years, but Gen AI with Data Cloud represents a significant advancement in capabilities and opportunities.
Broadly speaking, AI is a significant field with multiple subcategories. For this article, we’re going to simplify a bit and focus on predictive machine learning (ML) and Generative AI (Gen-AI). Predictive (or statistical) ML uses algorithms and models to make predictions about data. These predictions may inform a variety of business actions. Gen AI, on the other hand, creates new content (e.g., text, images, music) by learning and replicating patterns from existing data.
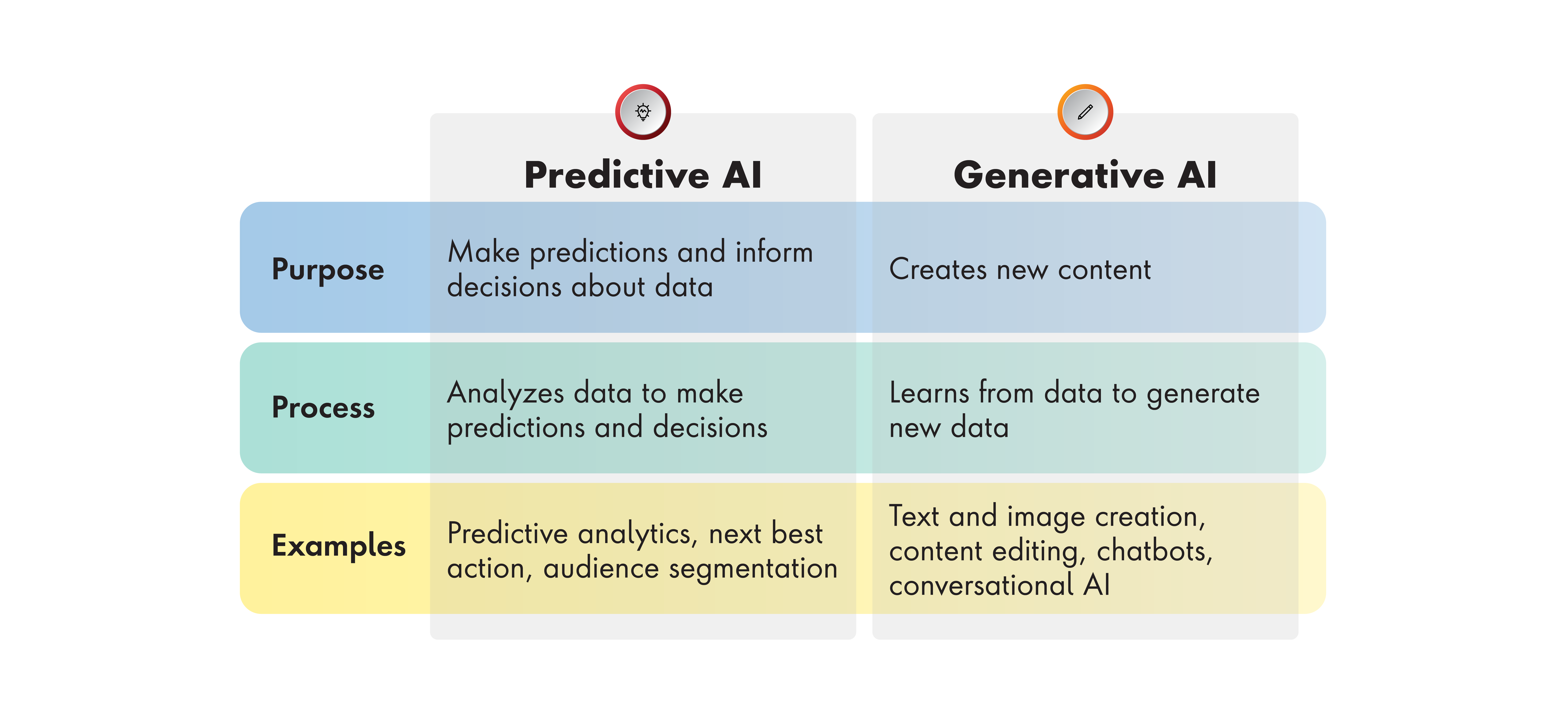
Salesforce is incorporating both Predictive ML and Generative AI into its applications, customer experiences, and user flow-of-work. This creates a number of new opportunities to both optimize how we use Salesforce and how our customers engage with our brands. However, to take advantage of this, we need to get more comfortable with Gen AI and how it works.
Gen AI fails when unconstrained prompts meet unconstrained expectations
Unconstrained prompts give the AI model more freedom to generate text, while constrained prompts provide specific instructions to guide the output. The choice between the two depends on the desired level of control and creativity in the generated text.
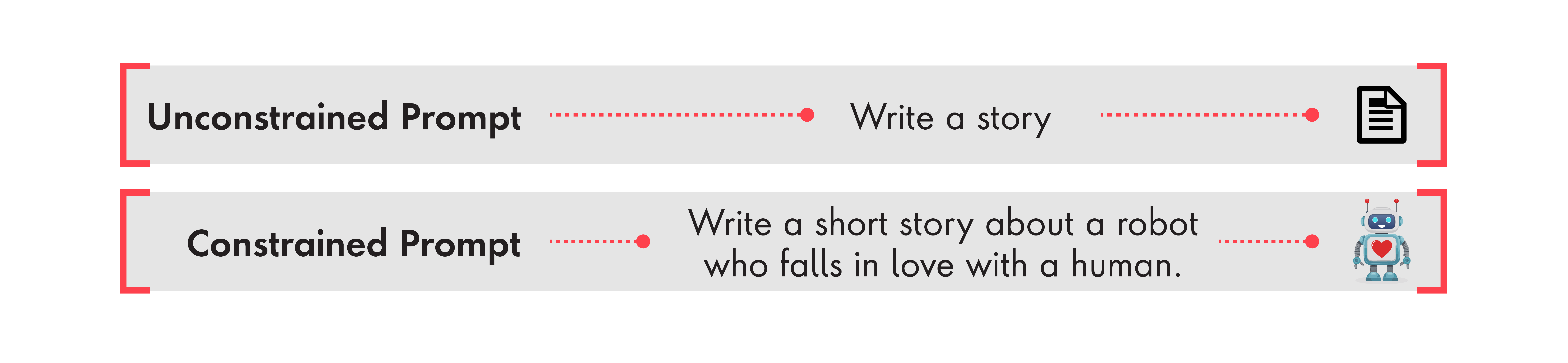
Key factors influencing Gen AI accuracy when prompted:
- Prompt Specificity: More specific prompts typically yield more focused responses, while broader prompts allow for more “creative”, but potentially less precise, outputs.
- Data Volume: Too much information can lead to confusion—concise inputs are often more effective.
- Response Guidance: Specifying desired style, format, length and level of detail often improves responses.
- Understanding LLM Capabilities: Managing expectations and recognizing AI limitations ensures more accurate and useful results.
“Grounding" is crucial for enhancing AI response accuracy and relevance. It involves applying context to AI outputs, significantly improving their usefulness. Salesforce has made this approach central to its AI strategy, offering multiple types of grounding that can be combined within a single prompt set. This feature makes Salesforce's AI solutions particularly compelling, as Salesforce often has access to the best customer datasets in an organization.
Salesforce offers three types of grounding:
- Field grounding: Directly references fields from Salesforce and Data Cloud in the prompt (e.g., customer record).
- Flow grounding: Incorporates data and process constrained within the process flow and its related context (e.g., most recent product orders combined with a question from a customer such as "When should I expect my order to arrive?").
- Document-based grounding: Enables incorporating unstructured information as part of a response (e.g., from a knowledge base).
These grounding techniques can be combined to create sophisticated prompts, allowing for highly contextual and relevant AI responses. Such advanced prompts can be utilized by both internal users and external consumers, enhancing the overall effectiveness and versatility of Salesforce's AI solutions.
Putting AI to work is a bit of art and a bit of science. The learning curve requires experimentation, and because of this, getting engaged now is imperative.
4 fundamental steps to the easy adoption of Salesforce AI
1. Identify and Categorize Use Cases
When identifying and categorizing use cases for AI adoption in Salesforce, consider the following approach:
- Start with inspiration from Salesforce's productized use cases. These have been developed into features, providing a solid foundation for implementation. Don't worry if your first attempt feels like a solution in search of a problem—this is a normal part of the process.
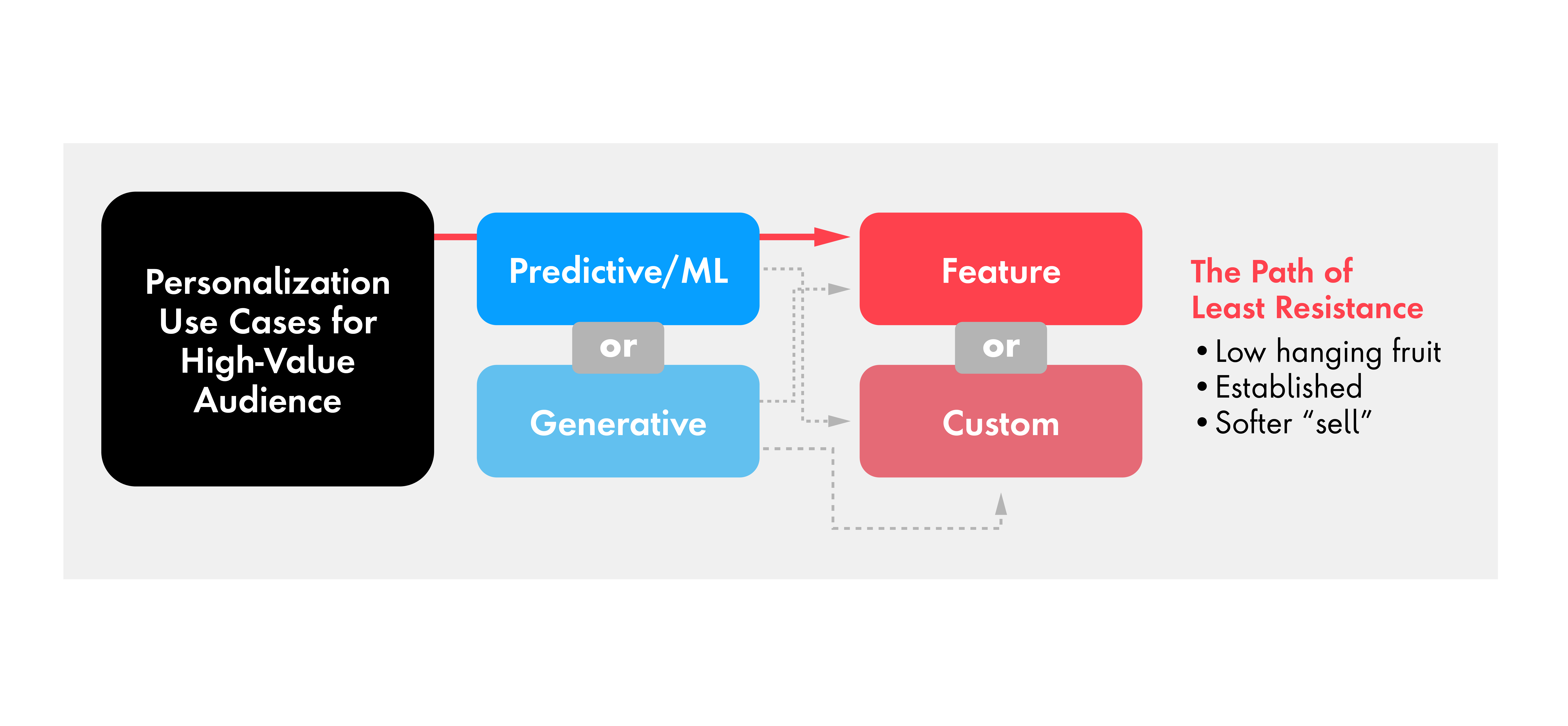
When considering specific applications, you have three main paths:
- Leverage existing Salesforce features: These are pre-built solutions designed to address common business needs.
- Implement custom Gen AI solutions: Develop tailored AI applications for unique business requirements.
- Utilize predictive or machine learning models: Apply data-driven forecasting and pattern recognition to enhance decision-making.
- Combine predictive machine learning with generative solutions: using your businesses’ data, you can create powerful predictions that inform generative capabilities.
For maximum impact, consider focusing on use cases that cater to high-value audiences within your organization or customer base. This approach ensures that your AI adoption efforts deliver significant value where it matters most.
2. Assess Data Readiness
Assessing data readiness is a critical step in adopting Salesforce AI solutions. This evaluation ensures your data foundation is robust enough to support AI-driven insights and predictions. Consider two key areas: Data Quality and Data Accessibility & Integration. Within each area, you’ll find specific factors that contribute to successful AI implementation.
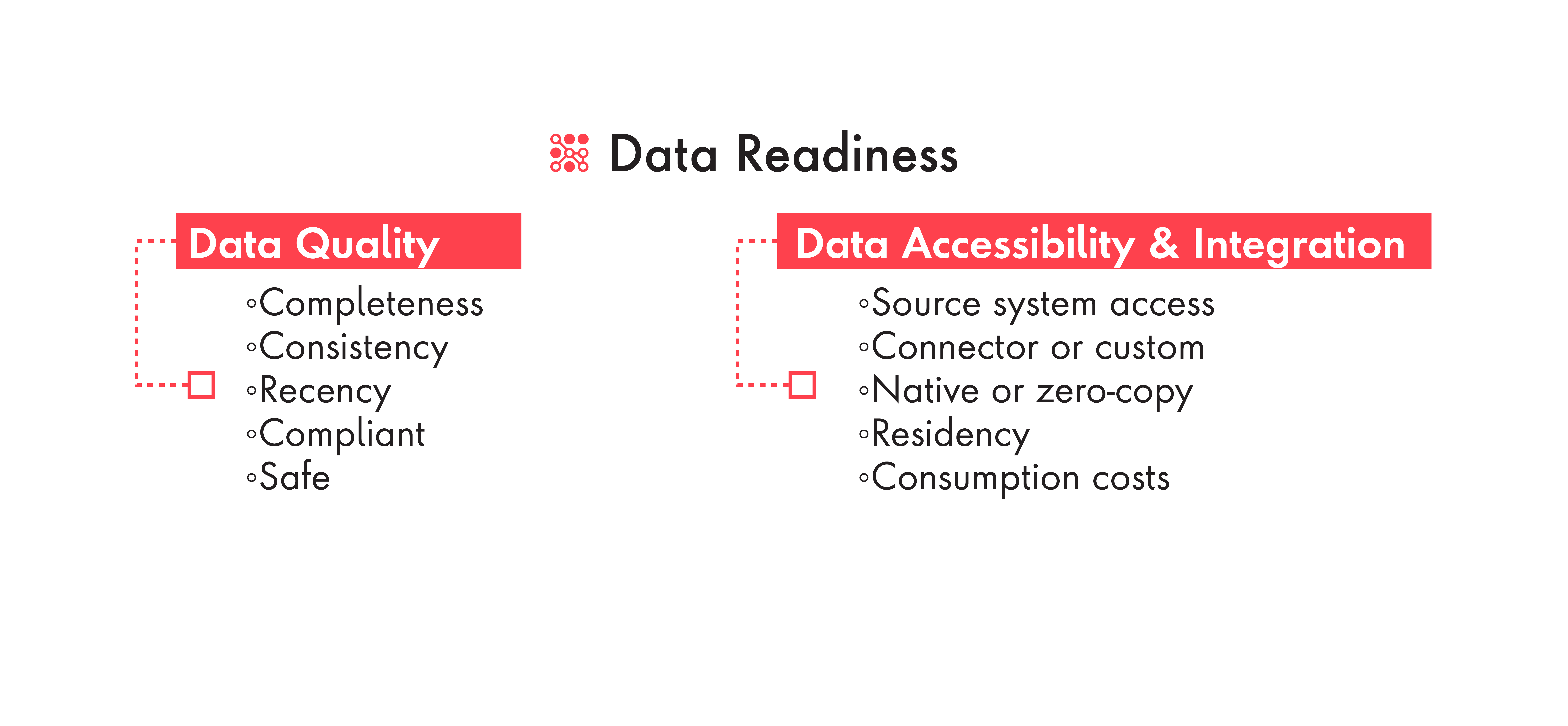
3. Plan for Governance
Effective governance is crucial for successful AI adoption. It ensures responsible use, manages expectations, and maintains data quality and accessibility. Consider the following key aspects:
Understanding and Education:
- Recognize varying levels of AI knowledge within your organization.
- Educate stakeholders on AI fundamentals, clearly distinguishing between predictive and generative AI applications.
Risk Management:
- Evaluate Risks: Assess potential risks associated with using AI, such as privacy violations or unintentional harm, and take steps to mitigate them.
- Implement Safeguards: Implement safeguards such as human oversight or explainability to ensure ethical and transparent AI behavior.
- Monitor Performance: Continuously monitor AI performance and make necessary adjustments to maintain accuracy and prevent unintended consequences.
Data Governance and Compliance
- Data Ownership: Identify and document data owners and stakeholders responsible for maintaining data quality and governance.
- Data Privacy and Security: Assess compliance with data protection regulations (e.g., GDPR, CCPA) and ensure appropriate access controls and encryption methods are in place.
- Data Stewardship: Define and enforce policies and procedures to maintain data integrity, accuracy, and availability over time.
4. Define a Pilot Program with Measurement
Launching a well-structured pilot program is crucial for successfully adopting Salesforce AI. When defining your pilot program, consider first adopting out of the box Einstein capabilities, and work up to more advanced custom predictive and generative solutions.
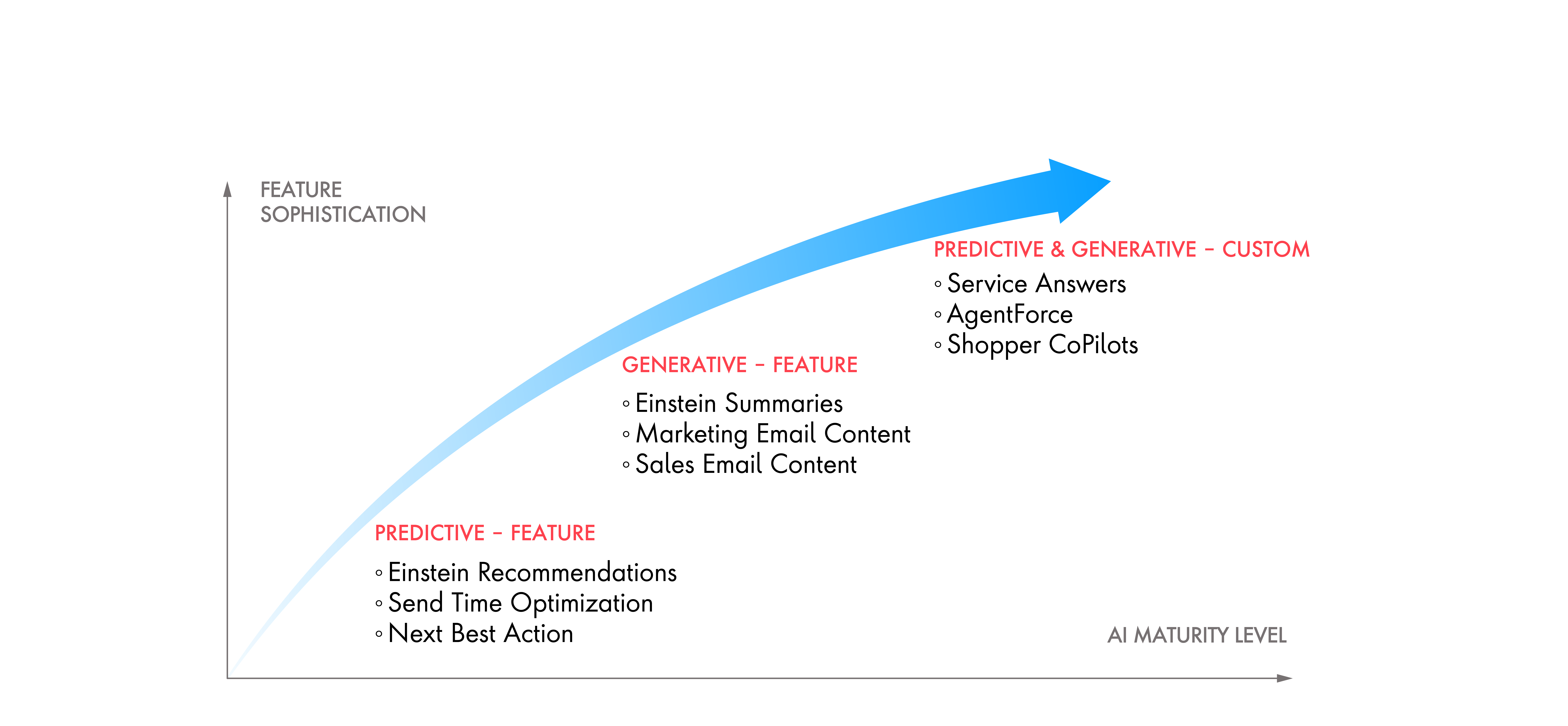
Key considerations for your pilot program:
- Select appropriate AI capabilities based on your current position on the maturity curve.
- Identify early adopters who can provide meaningful feedback.
- Implement an effectiveness measurement plan to quantify the impact of AI adoption.
A final note
Putting AI to work is a bit of art and a bit of science. The learning curve requires experimentation, and because of this, getting engaged now is imperative. Even if getting started is you, at home, conversing with ChatGPT, Claude or Gemini to plan weekend getaways or tonight’s dinner—that experimentation will help you understand more about how it works. At work, we need to plan with that mindset. AI isn’t a backlog item that can be checked off. Both the technology and how we apply it will be constantly evolving.
Let’s Connect
Reach out today to explore how Publicis Sapient and Salesforce can help your business unlock the full potential of AI. Learn more about our Value Alignment Lab—an outcome-driven discovery workshop focusing on delivering value.
Related Reading
-
Insight
The Future of Customer Engagement: Inside Salesforce's AI Ecosystem
Salesforce's innovative vision for data and AI enables revolutionary customer engagement experiences.
-
Insight
Unlock Real-World Value through Practical AI and Salesforce
Publicis Sapient is partnering with Salesforce and Publicis Sapient AI Labs to help businesses unleash practical AI solutions to solve complex challenges.
-
Insight
Publicis Sapient’s Value Alignment Lab
An outcome driven discovery workshop focusing on delivering value.