What issue can we solve for you?
Type in your prompt above or try one of these suggestions
Suggested Prompt
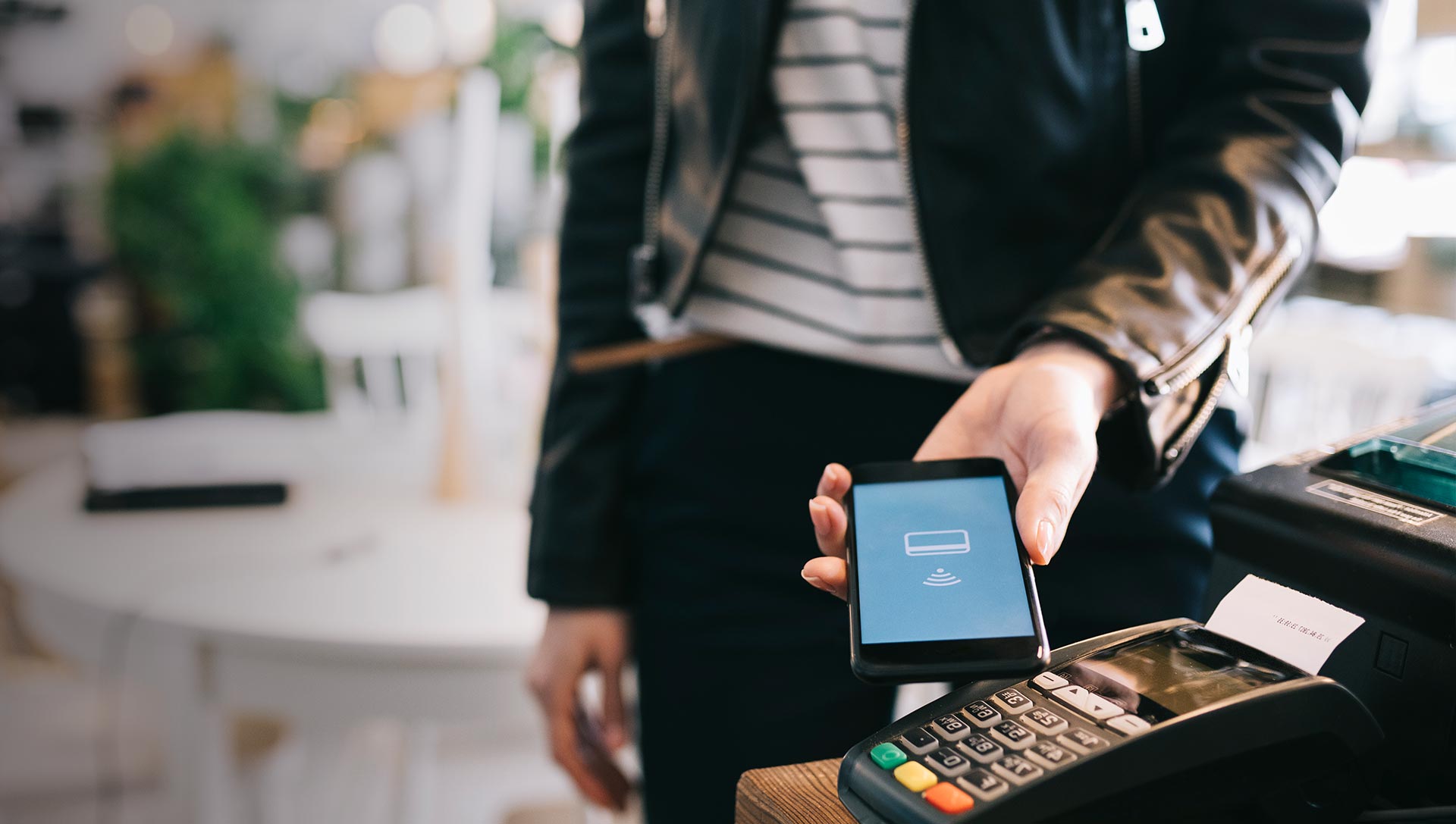
In the News
How Credit Card Issuers Can Gain from Machine Learning
Our Varun Mathur, Manager, Business Consulting, discusses the benefits of machine learning for credit card issuers via Mint.
Leveraging AI for enhancing credit cards value proposition as well as lending and payments functions now can reap several benefits.
India has witnessed a strong growth in credit card adoption – 39 million cards were in circulations at the end of June 2018, up 25% year-on-year. While consumers continue to see credit cards as a convenient way to extend their purchasing capacity, with the ongoing payments’ disruption, the plastic credit cards as we know today, may not be part of the payments’ future. Take the case of UPI 2.0, which allows merchants to leverage overdraft facility as well as block funds in the customer’s savings account for future usage, in many ways functioning like a credit card. Or online retailers trialing debit cards for EMIs and “pay-later” platforms that offer instant credit at checkout. Add to this the fast adoption of digital wallets that continue to push conventional payment instruments in the background. People may still link their wallets to credit cards, but issuing banks will lose some of their brand appeal and crucially, a direct access to customer spending data.
So how do credit card issuing banks navigate this scenario of consolidating rapid growth today while preparing for the future? The answer lies in adopting a more customer centric approach. If issuers are able to better predict consumer behaviour, they have a stronger chance of offering highly personalized solutions. Adoption of artificial intelligence and more specifically machine learning offers this opportunity. Several leading Indian banks are already experimenting with AI for improving customer service and optimizing backend processes. Leveraging it for enhancing credit cards value proposition and more broadly, lending and payments functions now can reap several benefits.
Benefits of machine learning for issuers
Machine learning can help issuers make better sense of the exponentially increasing data (both transactional and behavioural) and more accurately predict spending behaviour. Ultimately, this can lead to better customized experiences and drive top line and bottom line growth. Let’s now look at some specific applications of machine learning in the credit card value chain.
Risk assessment: Timely, accurate assessment of credit worthiness is vital as credit card applicants dislike the uncertainty around their applications. Conventional models of risk assessment rely on data sources such as existing debt burden and payment histories and deploy linear statistical modelling together with human judgement. However, with the growing volume and variety of data, machine learning algorithms can be more useful, enabling lenders to dynamically include additional sources such as current wealth, property ownership, insurance claims, social network profile and other personal indicators, thereby, creating a more holistic profile of the borrower. This approach also helps includes borrowers with weak credit scores. Already, Mumbai based start-ups, Rubique and CreditVidya leverage AI to innovatively assess credit worthiness, speed up decision making and expand reach for banks.
Effective targeting and retention: Consumers today have a problem of too many credit card options to choose from. Issuers can feed first and third party data to machine learning systems to come up with a target audience profile and the relevant channels to share most appropriate card options. The same principle can also be applied to timely target disgruntled customers and reduce churn.
Customer care: The advancements in machine learning are helping Natural Language Processing (NLP) applications such as chatbots become more contextual. Through chatbots, credit card issuers can ensure round the clock assistance across all stages of customer interaction including product selection, on-boarding, payments and usage. Smarter chatbots can also learn to detect irate human behaviour and accordingly escalate queries to customer care executives.
Personalized rewards: Rewards are most important reasons for keeping a credit card on top of the wallet. Machine learning can help issuing banks get a true understanding of each customer and follow it up with tailored reward experiences. American Express for example uses machine learning to recommend restaurants to its card members. HSBC in the US has experimented with predicting how customers are likely to use their reward points and accordingly market its reward programs more effectively.
Contracting fraud: Issuers bore more than 70% of the losses arising from global card fraud in 2016. Machine learning systems feeding on historical transaction and behaviour data can help identify patterns associated with fraud. This gives issuers a better chance to counter the ever evolving sophistication of cyber-attacks, compared to traditional rule based techniques.
New revenue opportunities: Machine learning capabilities can help banks open-up new monetization avenues. “Predictive spending insights”, built on transaction and third-party data is one such example. Case in point is American Express’s AmexAdvance, that combines transactional and third-party data to brand marketers and media partners deliver personalization services.
Implementing machine learning capabilities
Implementing machine learning is more feasible today because of the vast improvements in computing power, the abundance of data as well as storage and algorithmic advances. Like most other emerging technologies though, it is no panacea, and to get the most benefit, issuers need to build the right capabilities.
Develop an AI roadmap: Credit card issuers should clearly define the purpose, context and scope of why they would be using machine learning. In the short-term, issuers can focus on proven application areas such as fraud detection and over a period of time look at relatively more advanced use cases such as automated rewards recommendation. Moreover, issuers can also work with academia and technology partners to identify more futuristic use-cases.
Assess and build capabilities: According to a Harvard Business review analysis, companies that have strong experience in digitization have a 50% higher chance of generating profit from AI implementation. Issuing banks must therefore have a robust digital foundation, especially big data analytics and cloud capabilities. Finding the right talent, especially data scientists is also a big challenge and issuers can work with their technology partners or collaborate with fintechs to bridge the skill gap.
Stay on the right side of the regulation: Regulations such as GDPR have put entire onus on protecting customer data with businesses, imposing stringent fines for defaulters. Banks therefore must ensure complete compliance when venturing into data dependent application of AI. Equally vital is to communicate with customers on how their data is going to be used. This will also reinforce the trust consumers have in their brands.
Be cautious about bias: Decisions of machine learning algorithms can be prone to bias, one because they are designed by humans and second, because of the limited available test data. Any resulting bias, for example in the form a denial of credit card or inappropriate messaging may lead to regulatory sanctions and invite consumers’ backlash. Choosing the appropriate data samples that minimize bias is essential. Moreover, the insights generated by the system should be regularly monitored, especially because such systems learn over time and may offer unfavourable results in future.
In conclusion, credit card issuing banks must plan a systematic implementation of machine learning capabilities built on top of a solid digital foundation. This will go a long way in making the most of the growth today as well as in redefining the future of payments.