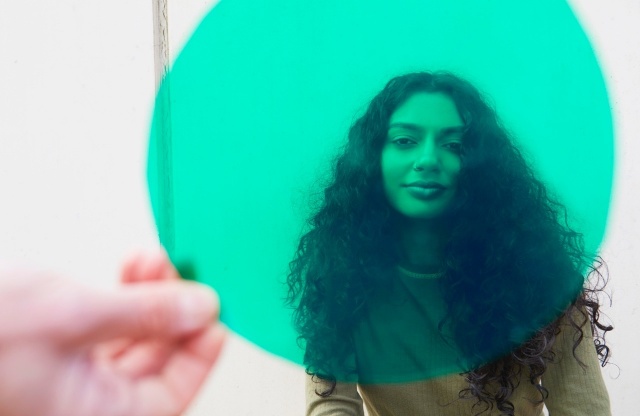
Insight
{{eyebrowContentTitle ? eyebrowContentTitle : activeTagName}}
Make it Personal Make it Personal Make it Personal Make it Personal
How AI and Machine Learning are Creating Custom Products and Experiences for the Masses
Type in your prompt above or try one of these suggestions
The Algorithmic Retailer
How traditional retail organizations can build competitive advantage through enterprise-wide data capabilities
A recent survey of C-suite executives revealed that six in ten had plans to increase their organization’s AI budget by more than 50 percent in the coming year. That sounds like progress—but is it?
As companies continue to grow their data capabilities, many large established organizations—traditional retailers among them—may be guilty of building and deploying AI solutions in a siloed way, segmenting efforts according to business function, as opposed to taking a holistic view of the organization. Working in this way compartmentalizes data, algorithms and insights by group, preventing organizations from unlocking the full value of their AI investments across the business.
On the other hand, taking an enterprise-wide, cross-function approach, organizations can improve the scalability of their AI efforts, creating value for the organization in the form of improved speed and accuracy, as well as operational efficiencies and cost savings.
These are the principles of algorithmic retail, a customer-centric platform that cuts across organizations silos to create enterprise-wide application of AI, machine learning, and other mathematical techniques to boost conversion and increase cross-sell and up-sell, while minimizing returns and shipping costs.
Algorithmic retail is a customer-centric platform that cuts across organizations silos to create enterprise-wide application of AI, machine learning, and other mathematical techniques to boost conversion and increase cross-sell and up-sell, while minimizing returns and shipping costs.
While many retailers are accustomed to using transactional data, it is less common for them to use contextual and customer data to inform business processes. Combining these elements greatly enhances the relevance and accuracy of the model.
Publicis Sapient worked with one organization to improve the accuracy of their demand forecasting process by incorporating cross-function and cross-channel data. Traditionally calculated at the showroom level with sales history, we added browsing and clickstream data based on IP location, which improved overall precision and accuracy, leading to:
Retailers can employ these same principles, particularly if they have a significant e-commerce platform. Even modest improvements in demand forecasting accuracy could present significant cost reductions. One model we produced showed that if a $10B retailer improved demand forecasting accuracy by as little as .25-.75 percent, they could realize a savings opportunity of $60M annually.
For most retailers, e-commerce is the fastest growing sales channel. It is also the least profitable. A big reason for that is a high rate of returns, with digital customers returning items up to three times as often as brick-and-mortar shoppers.
In addition to creating both inbound and outbound shipping costs, this negatively impacts multiple areas of the business, including mismanaged seasonal inventory, out-of-stocks, overstocks, refurbishment and repackaging—not to mention time. It takes 30 days to get a returned item reconditioned and ready to be resold.
An enterprise-wide approach to AI holds tremendous power to help realize this change, as models can take data from various points in the customer journey as well as cross-functional business metrics to predict return rates on various products, as well as for individual customers. In this way, organizations can address the risk of returns at the right point in the customer journey, either by offering incentives or deterrents to high-return customers, or by identifying needed updates to product descriptions or photos.
“We are on the brink of the algorithmic enterprise. Today’s generation of business and technology leaders can have a metamorphic influence on humanity by catalyzing applied AI for every enterprise.”
Rashed Haq
Related articles