What issue can we solve for you?
Type in your prompt above or try one of these suggestions
Suggested Prompt
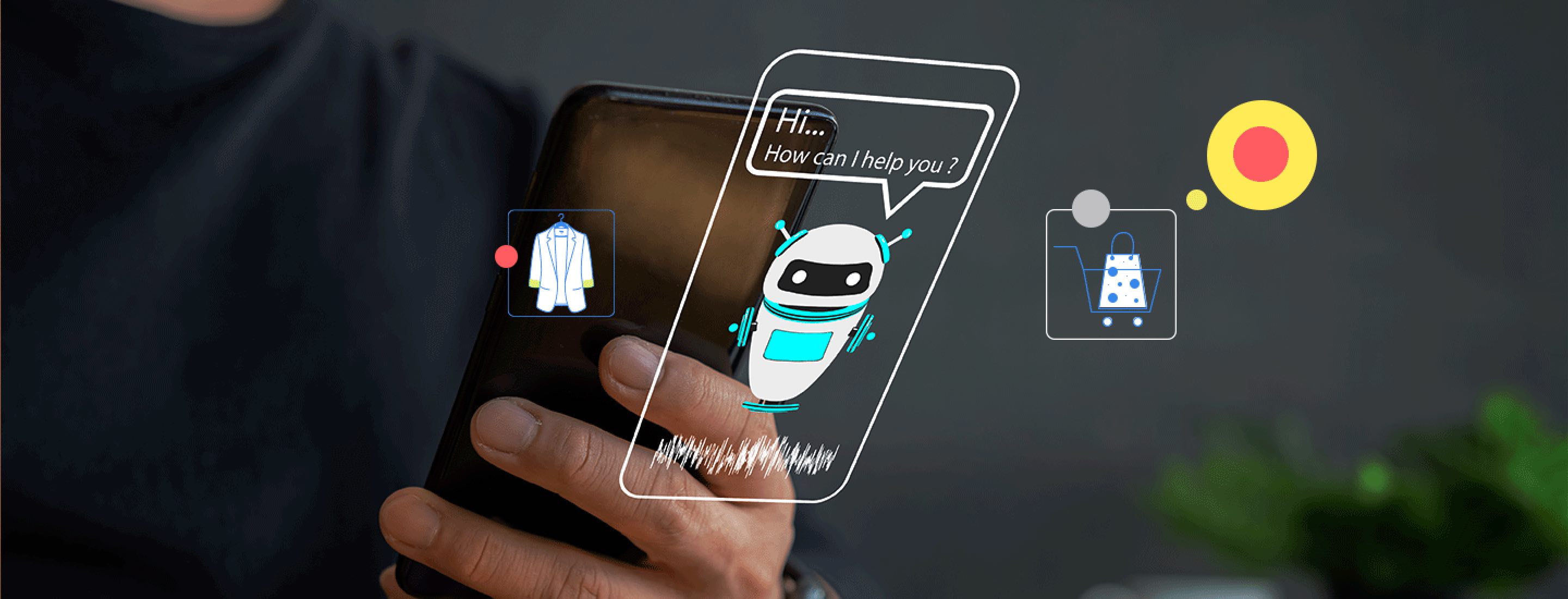
Insights
Beyond Product Recommendations: How Retailers Can Increase Profits with Generative AI
Beyond Product Recommendations: How Retailers Can Increase Profits with Generative AI
Our experts summarize the state of generative artificial intelligence in retail in 2024, including use cases across customer experience, supply chain and back-end e-commerce.
In this article:
- What is generative AI? Generative AI is a type of artificial intelligence model that can be trained to create new content based on a sample data set. The most advanced chat-based models, like ChatGPT’s GPT-4, can effectively mimic human conversation.
- How can AI be used in retail? Retailers can integrate generative AI to drive down costs in three main areas: customer experience, supply chain and back-end e-commerce.
- Which retail brands are using generative AI? Retail marketplaces like Walmart, Amazon and eBay have already implemented the technology for employee efficiency, customer experience and business capability building.
- What are the drawbacks? Early-stage adopters need to address the technology’s flaws, risks and limitations through focused experimentation to avoid potential disillusionment and consumer backlash.
- How is AI going to change the retail industry? Data strategy, a prerequisite for generative AI implementation, will become the greatest differentiator for e-commerce players in the next decade.
Generative artificial intelligence (AI) has the power to completely transform retail experiences, from the automated creation of online storefronts to personalized customer shopping journeys. But is now the time for retailers to embrace the nascent technology?
That’s the question that the retail industry is grappling with after recent advances in the area of generative AI or an artificial intelligence approach that can generate new content such as images, videos, audio, text and code that did not exist before. Taking advantage of this nascent technology is the perfect opportunity to promote growth for your business. Artificial intelligence has the capability to unlock maximum ROIs by analyzing large tables of data and identity areas of potential revenue that can increase profitability, or predict a new revenue source entirely. Utilizing AI undoubtedly improves efficiency and could cut unnecessary costs burdening profitability potential.
Advanced generative AI systems could optimize any function within the retail industry through conversational and creative abilities, including:
- Customer service
- E-commerce transactions
- Marketing creative
- Website navigation
- And much more
According to a global consumer survey, consumers are not only open to using generative AI tools themselves to improve their e-commerce shopping experiences, but they’re excited about them.
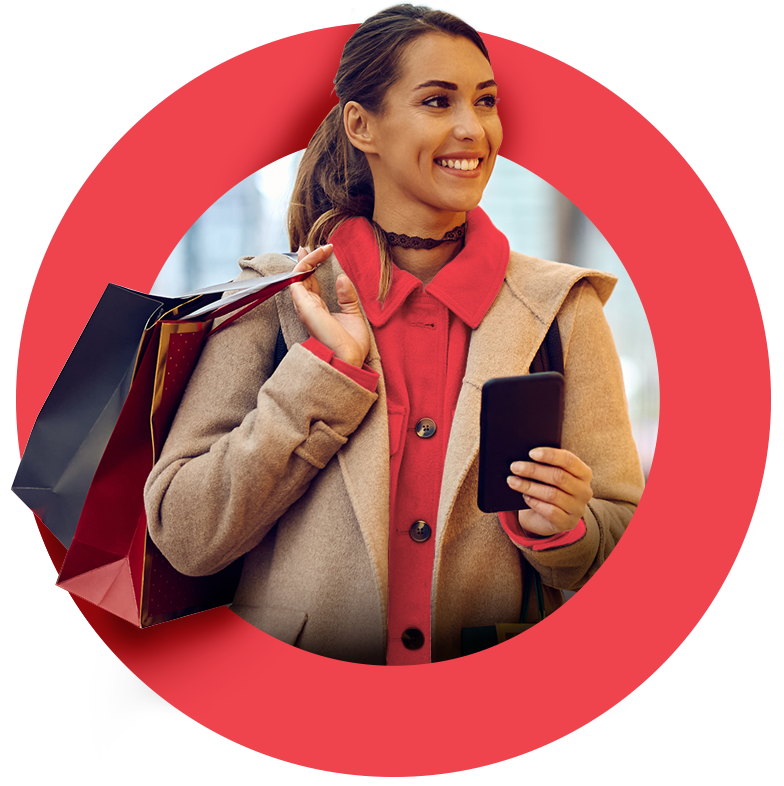
Neiman Marcus Group’s AI Success
Tune into our conversation with Neiman Marcus Group’s VP of Information Technology to discover insights on leveraging Generative AI as a catalyst for marketing transformation, enhancing customer experience and operational efficiency to unlock growth.
87%
of shoppers that have used a generative AI tool are excited about something generative AI will bring to their shopping experience
27%
of shoppers are excited about generative AI’s ability to improve real-time price comparison and deal alerts, as well as overall online search results
25%
of shoppers would use a virtual generative AI shopping assistant if it were available for online shopping
40%
of shoppers that have used a generative AI tool already say the same
However, integrating conversational AI into workflows and customer journeys presents a steep learning curve for employees, customers and brands alike. The flaws and risks of the emerging technology, including inherent biases, lack of consumer trust and factual inaccuracies, will require time and effort from retailers to combat.
“How can we use a technology like this to catapult businesses into the next area of growth and drive out inefficiencies and costs? And how can we do this ethically?”
Sudip Mazumder , SVP and Retail Industry Lead
We asked Publicis Sapient retail and AI experts where retailers can integrate the technology right now and what leaders need to know about its capabilities and limitations.
What generative AI is—and what it isn’t
Generative AI is a broad term used to describe artificial intelligence models that can generate new data samples that are similar to training data. Open source models like GPT-4 and Google’s Bard can write poetry or informational articles, create trip itineraries, give life advice, take tests and answer any prompt or statement you throw at it, utilizing data sets as large as the entire internet as a source.
Despite the initial breakthrough of ChatGPT’s large language model, the power of generative AI goes far beyond chatbots. Advanced generative AI models have the power to create infinite, contextualized content of any format. Studies show that humans already can’t tell the difference between text, code and images created by artificial intelligence and content created by people.
Generative AI uses probability, not human-like reasoning
However, generative AI systems like GPT-4, the model used to create the ChatGPT application, don’t generate text based on logical reasoning or human intelligence. They’re simply generating the most likely “correct” responses based on the context defined by the available data set, i.e., the logical next step in the sequence.
For example, if a retailer used AI to create a series of personalized advertisements across different customer demographics, without further programming, the generative language model would simply use a probability distribution to predict what should come next based on the prompt and generate a plausible-looking advertisement.
While these limitations prevent generative AI from running marketing departments anytime soon, there are still several retail applications that are ripe for the most impressive aspect of the technology: thepower of human-like prompt continuation.
How retailers can take advantage of generative AI
Conversational commerce: generative AI and the customer experience
Generative AI is quickly changing the way customers interact with retailers online.
“The modality of online shopping interactions, and e-commerce interfaces themselves, may soon change,” says Sara Alloy, head of retail experience at Publicis Sapient. “You’re going to see a much better quality of search with more tailoring, customization and efficiency.”
Conversational product search
While most shoppers can use search bars to find the products they’re looking for, conversational commerce (powered by generative AI) accelerates the search process, potentially increasing conversion rates and average basket sizes for retailers.
Brands are implementing A/B tests of conversational product search bars to assist customers in finding specific products more efficiently, like searching for all of the ingredients in one recipe through a question or asking about each item of clothing in a full outfit.
Chatbot support
Customer service chatbots powered by generative AI can reduce staffing needs and support agents by providing complex and engaging responses. While many chatbots currently have only 15 or 20 decision trees, advanced generative models open the potential for chatbots with infinite paths of conversation.
Retailers also have the opportunity to play around with conversational styles that match their brand and personalize interactions for customers, changing the negative perception of automated chatbot features.
Cross-selling and upselling
Finally, generative AI can provide more intelligent shopping suggestions based on search history and other customer demographic data. While retailers currently use analytics and tags to monitor and enhance consumer experiences, generative AI could more automatically suggest the next logical purchase or step in a customer journey, without manual journey design.
Automated content generation: generative AI and back-end e-commerce
Not only can generative AI improve the front-end customer experience, but it can also automate workflow on the back end. While creative work from generative AI models lacks complexity and nuance, new systems can easily automate simple, consistent content tasks at a human level.
“Generative AI can speed up content creation for commerce,” says Rakesh Ravuri, CTO at Publicis Sapient. “Future iterations of these models will hopefully provide more transparency and fewer errors, but the information still needs to be reviewed and validated.”
There are several scenarios within back-end e-commerce transactions where generative AI is already assisting with content creation:
Consistent product descriptions
In the past, retailers invested in AI to automatically A/B test product descriptions, finding the most engaging variation. However, recent advancements in AI’s contextual ability allow retailers, specifically retail marketplaces, to automatically standardize descriptions across a variety of sellers.
Often, third-party vendors upload wildly inconsistent product descriptions. Rather than hiring associates to manually rewrite product descriptions, content writers can directly prompt generative AI with specific instructions and constraints to create product descriptions that are standardized, grammatically correct and in line with brand tone.
In a similar manner, generative AI can be employed to collate and analyze product reviews, providing retailers with valuable insights into customer sentiment and product performance. This allows for proactive adjustments to product listings and marketing strategies, further enhancing the customer experience.
Personalized product images
Another formerly manual content creation process that can be improved through generative AI is product images. High-quality product images require photographers, graphic designers, models and creative staff for the shoot. Generative AI would allow retailers to generate personalized product images for each customer based simply on text descriptions and historical image data.
For example, an athletic apparel retailer could automatically generate an image of a college student wearing a sports jersey on a college campus backdrop for a 19-year-old customer. If customers are willing to provide more personal data or even their own prompts, generative AI could show the customer each product in a variety of different contexts of their choosing—even on a digital twin of their own body.
Auto-fill transaction flows
The same use case could also apply more generally to full web pages, allowing retailers to guide their vendors and customers through the purchase journey even faster. Right now, most e-commerce website flows are generic and fixed or based on simple inputs like time zone or channel.
Generative AI would allow retailers to provide abbreviated site experiences for each customer and each vendor, automatically filling in product, store or customer information on the back end.
Secondary decision-making: generative AI and supply chain optimization
What about use cases outside of the consumer interface? There are a variety of human-to-human and human-to-machine interactions that could be enhanced and streamlined through the conversational ability of large language models
One use case that’s less explored in the retail space thus far is the supply chain. Generative AI as a communication vehicle could reduce costs and create more seamless experiences for supply chain leaders, specifically through accelerating secondary decision-making.
“We have supply chain control towers for visibility and tracking. We also have proper prediction and forecasting algorithms that use AI,” says Ravuri. “Generative AI could add a layer of decision-making support to existing technology for a variety of unique contexts.”
There are several scenarios where generative AI could add to current supply chain technologies:
Where is my package?
The biggest question in supply chain is always: “Where is the package?” Currently, stakeholders have to reach out manually or use limited tracking information to access package touchpoints across a variety of different databases.
Generative AI with conversational capabilities could answer this question much faster without room for error or confusion. For example, a customer or associate could ask a chatbot specifically, “When will the package get to me?” or “Can the package be rerouted?” and generative AI could resolve these simple questions interactively.
Packing configurations in transport
Supply chain managers currently optimize packing configurations using AI-powered algorithms, but generative AI could provide a trouble-shooting layer for unique contexts. For example, something may be leaking from the floor, or managers may prefer a configuration with a one-meter space for people to walk in. A manager could ask generative AI for a solution to a specific packing scenario using simple language.
Generative AI also has the power to quickly determine new configurations for shipping labels. Introducing new elements to this critical real estate is often a complex decision-making process based on unique variables with lots of room for error and inefficiency.
A large language model could generate new label printing combinations without violating any constraints to assist decision-makers in finding the best possible use of space.
Learn more about common pitfalls when implementing AI in the supply chain and how to ensure success.
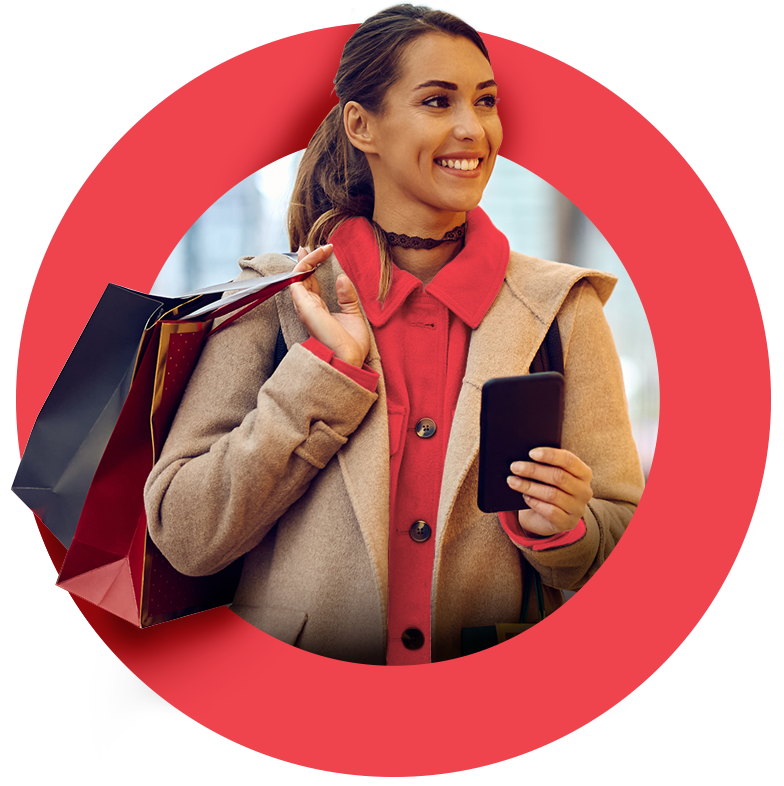
Neiman Marcus Group’s AI Success
Tune into our conversation with Neiman Marcus Group’s VP of Information Technology to discover insights on leveraging Generative AI as a catalyst for marketing transformation, enhancing customer experience and operational efficiency to unlock growth.
Which retailers are ahead of the generative AI curve?
While most retailers are still experimenting with generative AI or creating an AI strategy, some retailers have already rolled out generative AI tools, policies and experiences.
Here are some of our experts’ favorite real-time examples:
Associate efficiency: Walmart's internal AI-powered personal assistant for remote workers
“While it began as an experimental generative AI “playground” for remote workers, Walmart Canada’s generative AI tool “My Assistant” has officially rolled out as a collaborative chatbot across the company. The technology is meant to help associates simplify tedious tasks, like taking meeting notes or summarizing large documents. In the future, the retailer hopes to integrate My Assistant with the company’s intranet and HR platforms as a self-service portal that can offer on-demand information to all employees.”
Sudip Mazumder, Senior Vice President and Retail Industry Lead
Customer experience: Amazon’s AI-generated customer review summaries
“Leveraging the power of customer feedback, Amazon has introduced an AI-powered feature that generates concise summaries of product reviews, enabling buyers to make informed decisions without sifting through a sea of text entries, star ratings and customer photos. This feature distills the essence of customer reviews, highlighting key themes and extracting valuable insights from repeated phrases. With a few clicks, customers can delve deeper into specific aspects of the product, guided by the AI-generated summary. Amazon's vast collection of reviews has transformed it into a major resource for customers, regardless of their purchase intention. This feature holds immense influence on consumers, and reducing review fraud through technology will only amplify its impact.”
Jackie Walker, Senior Director of Retail Customer Experience
Customer experience: eBay’s product description generator for third-party sellers
“eBay is leveraging generative AI to streamline the product listing process for third-party sellers. By analyzing product photos, AI can automatically generate comprehensive descriptions, including titles, product details and release dates. This innovation significantly reduces the time and effort required for sellers to create product listings, although the feature is still in the early stages.”
Sara Alloy, Head of Retail Experience
Capability building: Walmart’s commitment to use AI ethically and responsibly
“Walmart's Responsible AI Pledge outlines six key principles for ethical AI development and deployment, aiming to ensure AI is used responsibly and beneficially while safeguarding customer trust and privacy. Walmart is the first retailer to publicly release guardrails in this manner, encouraging other retailers to adopt similar principles.”
Kevin Drummond, Managing Director
The drawbacks of generative AI for retailers
Despite the successful examples above, rolling out generative AI processes and experiences in the early stages of the technology poses a number of risks and challenges that retailers should be aware of.
The creator of ChatGPT previously said it was a “mistake to be relying on it for anything important right now” due to its propensity to provide strikingly believable yet nonfactual answers without the ability to vet or validate them—and it takes significant investment to code in this capability.
ChatGPT’s creator also noted that “regulation will be critical and will take time to figure out.” Retailers looking to develop their own generative AI models before industry regulation will need to systematically teach AI literacy and create ethical policies for their associates to avoid consumer backlash from generative AI gone wrong.
“Brands need to be very transparent with people about when they’re communicating with AI and make those choices wisely,” says Alloy. “We’ve already seen public outrage over inappropriate usage of the technology in sensitive situations.”
How generative AI will change the retail industry
While the initial hype surrounding generative AI may have subsided, this classic decline in fanfare around new innovations should not be misconstrued as a lack of enduring value or relevance for the technology.
In fact, the opposite is true—in several years, generative AI could become the cornerstone of e-commerce experiences.
Retailers should be prepared for this future, whether they choose to integrate generative AI into their own e-commerce stores and businesses or not.
What’s next?
Data will equal dollars
The actionable takeaway for retailers should be investing in data strategy. Generative AI models rely on large, unstructured data sets, and the quality of this data has a direct impact on the ROI of the model itself. For retailers, much of this data will come from customers—across channels, regions and brands. In order to collate this data, retailers need to:
- Create and centralize customer data using a robust internal data strategy and governance
- Invest in tech talent or upskilling associates to work with artificial intelligence tools, whether it’s to create efficiency with content creation or build out proprietary large language models
- Rethink legacy technical architecture to accommodate generative AI, including CMS systems, supply chain mechanisms and customer data platforms
Retailers that invest in their data inputs (customer data, data quality control, data infrastructure, etc.) will quickly gain traction in the AI space over retailers that jump to their data outputs. Retailers can also utilize this data to analyze market trends and customer feedback that could be used as an opportunity to identify new revenue sources.
Getting started with generative AI
So, for retailers that are looking to test and learn with their first use cases, what’s the best area of the business to start with?
Most should begin with conversational commerce.
“It’s the first inroad to test out this technology, before expanding it to other areas of your ecosystem,” says Mazumder. “Beyond that, retailers can prioritize new use cases by asking themselves the following questions.”
- Where are sales associates spending most of their time?
- What are the biggest missed opportunities to upsell or cross-sell?
- How can associates access critical internal knowledge more efficiently?
- Where are customers and sales associates experiencing the most frustration?
- What are the most frequently asked customer questions?
“Retailers should start experimenting now because this technology has the potential for a serious uptick in customer engagement and revenue.”
Sudip Mazumder , SVP and Retail Industry Lead
Overall, generative AI in the retail space is a long-term value play—requiring deep technical expertise across many subject areas.
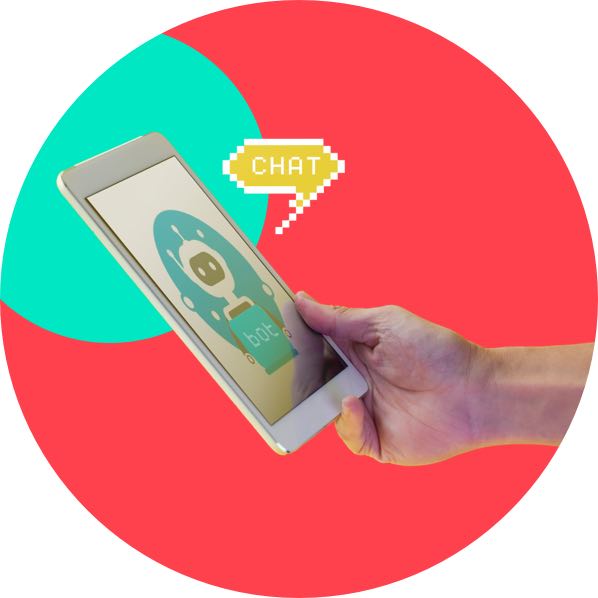
Create a Generative AI Retail Strategy With Publicis Sapient
Publicis Sapient was named a 2023 Market Leader in Generative Enterprise Services (Gen AI) by HFS Research, and can set up AI incubators in partnership with clients, powered by our unique SPEED formula. This approach combines strategic growth and business value, modern digital product thinking, next-generation customer experience, engineering and data and AI, all while leveraging our premier partnerships with Microsoft, Google and OpenAI.