What issue can we solve for you?
Type in your prompt above or try one of these suggestions
Suggested Prompt
The High Cost of Bad Data: What AI-Ready Really Means
Is data holding your AI plans back?
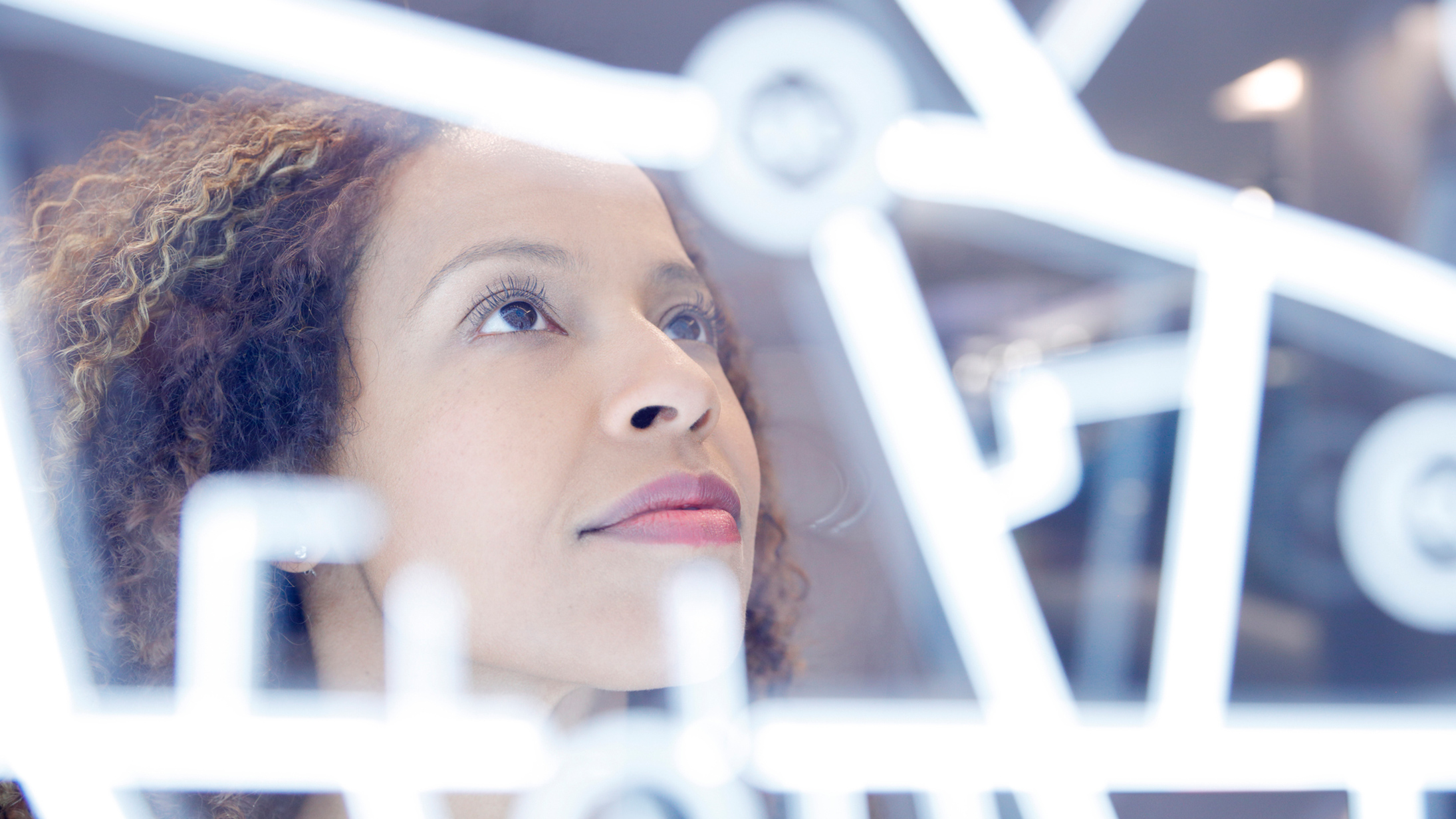
Executive summary:
- Even technically sophisticated organizations struggle with AI-ready data—our experts reveal that data estates are often "wildly immature" despite robust technical capabilities, creating a competitive divide between AI leaders and laggards.
- The journey to AI-ready data has three distinct phases: collecting and organizing your data, establishing clear AI-ready quality standards and implementing sustainable governance practices to maintain data integrity over time.
- Well-structured data delivers substantial business value even without AI implementation—from operational efficiencies to cost savings, organizations report 30 percent+ improvements in marketing ROI and significant supply chain optimizations from data readiness initiatives alone.
In this article:
The current state of enterprise data readiness
The three phases of AI data readiness
The business case for AI-ready data
Why invest in AI-ready data now—even if you're not using AI yet
Common challenges in preparing AI-ready data
How to start making your data AI-ready
Imagine this scenario: Your marketing team implements Adept AI, a sophisticated predictive analytics platform designed to optimize advertising inventory across your digital and traditional channels. The proof of concept was flawless—using a carefully curated dataset from your top-performing region, the system generated revenue forecasts with 97 percent accuracy and identified previously undetected patterns in audience behavior that could increase ad placement efficiency by 22 percent.
Excited by these results, you fast-track full production implementation across all business units. Six months and $2.3 million later, the project is in crisis. The AI confidently makes inventory predictions that miss targets by 35 to40 percent. Campaign optimization recommendations show no measurable improvement over your previous manual process. The same tool that seemed revolutionary in the pilot is now generating actively harmful business decisions.
What went wrong? The AI wasn't the problem—the data wasn't ready.
"Many companies we work with face similar situations," explains data expert Toby Boudreaux. "Parts of their ecosystem are still tracking data using CSV files on people's desktops that get emailed around. Despite being multi-billion-dollar global businesses, their average data infrastructures are fundamentally incompatible with enterprise AI implementation."
The proof of concept succeeded because it used a small, clean dataset from their best-performing region—data that had been meticulously prepared specifically for the pilot. But when scaled to production, the AI encountered fragmented data sources, inconsistent formatting, duplicate entries and critical gaps in historical information. The tool performed exactly as designed—but the data it processed wasn't remotely ready for AI utilization.
This disconnect between AI ambitions and data reality is shockingly common. According to experts, the gap between organizations with truly AI-ready data and those without is widening—creating a significant competitive divide between companies that can successfully leverage AI and those that cannot.
"No one's perfect on data, especially for AI, unless they've already gone through the process," says Rajiv Totlani, data engineering expert at Publicis Sapient. "I think every organization has some sort of data hygiene they can engage in."
What is AI-ready data?
AI-ready data isn't just a technical specification—it's a strategic asset that fuels your organization's AI capabilities. But what exactly makes data "AI-ready"?
According to our data engineers, AI-ready data encompasses several critical characteristics:
- Clean and accurate: Free from errors, inconsistencies and duplications that could lead AI models astray
- Relevant: Aligned with your business objectives and the specific AI use cases you're targeting
- Well-structured and organized: Formatted consistently and accessible through efficient systems
- Properly labeled: Tagged with appropriate metadata that helps AI understand context and relationships
- Well-governed: Supported by clear processes for quality control, lineage tracking and version management
"When we think about AI-ready data, it's data that's good data, which means it's clean and it's relevant to whatever the objectives of the organization are," explains Totlani. "It's structured and organized and housed well. That means it's easy to access. It makes sense how it's set up."
The current state of enterprise data readiness
Despite investing heavily in data collection and storage, many enterprises remain unprepared for effective AI implementation. Even technologically sophisticated organizations with robust development practices often maintain surprisingly immature data estates.
"A lot of companies and clients who are very sophisticated technically, with really robust guardrails, safe deployments, safe development—their data estates can very often be wildly immature," observes Toby Boudreaux,
The consequences are severe: organizations with poor data foundations struggle to generate meaningful insights or build effective AI models, regardless of the sophistication of their AI tools or the talent of their data scientists.
The three phases of AI data readiness
Transforming your enterprise data into a truly AI-ready state requires understanding the complete data lifecycle. Experts identify three crucial phases:
1. Getting your data ready
This initial phase focuses on:
- Collection: Gathering all relevant data from across your organization
- Validation: Ensuring accuracy and completeness of data
- Organization: Creating efficient storage and access systems
"If there's data that is like well structured, efficient to access and clearly labeled, that's also required for AI," explains Boudreaux. "It generally should make the business more efficient, even if it's not utilizing AI."
Â
2. Defining AI-ready standards
Once your data is collected and organized, you need to establish the characteristics that make it truly AI-ready:
Cleanliness: Removing errors, inconsistencies and outliers
Structure: Formatting data consistently with clear relationships
Labeling: Tagging with appropriate metadata for context
Relevance: Ensuring alignment with business objectives
"It's good to have clean, organized, well-maintained data that's labeled and structured properly," says Totlani. "Even if you're not looking to immediately get into the AI space, it's still good to get ready because it should make your business better."
Â
3. Maintaining data quality
The final phase focuses on sustaining and improving data quality over time:
Quality control: Implementing feedback loops and quality reporting
Governance: Managing updates, version control, security and monitoring
Auditing: Regularly reviewing data for continued accuracy and relevance
"Data governance is a whole sort of different domain and craft," Boudreaux notes. "It's about just really understanding your data and knowing the quality of it, being able to measure the quality of it, being able to manage access and literacy, issue resolution, stuff like that."
The business case for AI-ready data
Investing in AI-ready data delivers multiple benefits beyond just enabling AI projects:
Operational efficiency and cost savings
Even without implementing AI, clean, well-structured data improves business operations and decision-making. A financial services company saved "close to a couple hundred million dollars in engineering costs" by modernizing their data architecture to be AI-ready.
Â
Marketing and customer engagement
A retail organization achieved "significant percentage lift—30 percent plus" in marketing ROI by using clean data and AI to automate segmentation and campaign optimization.
Â
Supply chain optimization
In the automotive sector, AI-ready data has transformed inventory management. "For car dealerships and OEMs, we were able to better predict what car types and models should be utilized in certain regions," explains Totlani. This resulted in decreased lot time and reduced excess inventory.
Why invest in AI-ready data now—even if you're not using AI yet
You might wonder: If your organization isn't implementing AI immediately, why prioritize AI-ready data now? The experts offer compelling reasons:
Future-proofing your organization
"If somebody suddenly asked me to run a marathon tomorrow, if I don't run at all, that's awful," explains Totlani, using an analogy. "If I kind of run regularly now, it's like, OK, well, maybe I'm not going to do so well, but I might be able to finish."
By preparing your data now, you'll be ready when opportunities or competitive pressures make AI implementation urgent.
Â
Immediate business benefits
Well-structured, clean data delivers value regardless of AI implementation:
- More accurate reporting and analytics
- Improved operational efficiency
- Better decision-making
- Reduced data management costs
"It should make your business more efficient, even if it's not utilizing AI," notes Totlani.
Common challenges in preparing AI-ready data
Organizations typically face several obstacles when making their data AI-ready:
Data silos and integration issues
"In the FS sector, financial services—their data, although they kind of had all their data—it was just either very outdated in extremely different areas," explains Totlani. "Some data is housed in certain types of tables. Other data is housed in maybe a different software or a different vendor, different types of structured data."
Â
Poor data governance
Many organizations lack clear processes for maintaining data quality. "If you found some quality issues but you don't have the governance side... you get a bad outcome, and you don't know the lineage because data governance is a whole sort of different domain," notes Boudreaux.
Â
Balancing structure and flexibility
Too rigid a structure can limit the value of your data, while too little structure makes it unusable for AI. "If you have well-structured stuff and it's well-governed, you're kind of in the ideal starting state," says Boudreaux.
How to start making your data AI-ready
Based on expert insights, here's a practical approach to begin your AI data readiness journey:
1. Assess your current data state
Begin with an honest evaluation of your data ecosystems:
- What data do you currently collect?
- How is it structured and stored?
- What quality control measures exist?
- What barriers prevent optimal data utilization?
Â
2. Prioritize based on business impact
Not all data needs to be AI-ready immediately. Focus on high-value areas:
- Which datasets support critical business functions?
- Which would deliver the most value if optimized?
- Where would improved data quality immediately impact operations?
"It varies a lot on the end goals and use cases that you're trying to solve," Totlani explains. "I think data organization and how the data interacts together, how it's accessible—figuring out how we make sure that all of our data is easy to access and easy to connect through those different data sources is more important than even what data to focus on."
Â
3. Implement incremental data governance
"Incremental data governance...is sort of like how your doctor would approach your health," explains Boudreaux. "You don't have to get everything perfect, but let's do something today. Let's get a little bit better."
Start with manageable improvements:
- Create data dictionaries and catalogs
- Establish data quality standards
- Develop consistent naming conventions
- Implement basic quality checks
Â
4. Build cross-functional collaboration
Data readiness isn't just an IT responsibility—it requires organization-wide commitment:
- Engage business stakeholders in defining data requirements
- Establish shared data quality standards
- Create cross-functional data governance teams
- Develop common data literacy across departments
The scientific nature of AI and data quality
Â
Unlike traditional software development, AI implementation follows a scientific method—meaning outcomes aren't guaranteed, and experimentation is essential.
Â
"It's called data science for a reason," Boudreaux emphasizes. "There is a scientific method, and if you follow it strictly, you can get somewhere, but it takes more time and more creative thinking."
Â
This scientific approach means:
- You need to form hypotheses about what data will be valuable
- Testing and validation are critical
- Feedback loops must be established to improve data quality
- Results may not be immediately predictable
Â
"If you do something, if you train a model, and it takes four hours...you can't know if it was a good pass until you're at the end of it and you can validate it. And if it's a bad pass, you basically have to do the whole thing again," Boudreaux explains.
Â
The future of AI-ready data
As AI capabilities advance, the standards for AI-ready data will continue to evolve. Forward-thinking organizations should prepare for:
Vector databases and embeddings
"There's a type of database called a vector database that's very important in the LLM world," explains Boudreaux. These specialized databases store numerical representations of data that help AI understand relationships and similarities.
Â
Self-improving data systems
AI itself will increasingly help manage data quality. Systems that can automatically detect anomalies, standardize formats and identify data gaps will become standard components of AI-ready data infrastructure.
Â
Proprietary data as competitive advantage
"Large enterprises have a significant moat—proprietary corporate data, knowledge assets and expertise," notes one of the experts. As public AI models become more widely available, your unique, high-quality proprietary data will increasingly become your competitive differentiator.
Conclusion: The strategic imperative of AI-ready data
The journey to AI-ready data isn't merely a technical exercise—it's a strategic business imperative. Organizations that successfully transform their data foundations will find themselves equipped not just for current AI applications but for future innovations yet to be imagined.
As Totlani concludes: "Having clean, organized, well-maintained data that's labeled and structured properly—even if you're not looking to immediately get into the AI space, it's still good to get ready and have that data ready for AI because it should make your business better."
By investing in AI-ready data today, you position your organization to thrive in an increasingly AI-driven business landscape, where the quality of your data may ultimately determine your competitive success.
Related Reading
-
Insight
Top 5 Ways to Protect and Secure Data in the Age of AI
In the age of AI, data security isn't just a priority—it's a necessity. Stay ahead of the curve and safeguard your organization's most valuable asset.
-
Service
Data & Artificial Intelligence Services
Unlock transformative growth with innovative data and AI solutions at Publicis Sapient
-
Insight
Omnichannel Success Starts with Smart Data
What’s the key to an impactful omnichannel strategy? A robust data ecosystem.