What issue can we solve for you?
Type in your prompt above or try one of these suggestions
Suggested Prompt
The Artificial Brain: How Agentic AI Workflows Reshape Business Execution
What they are, how they work and how they could change your life
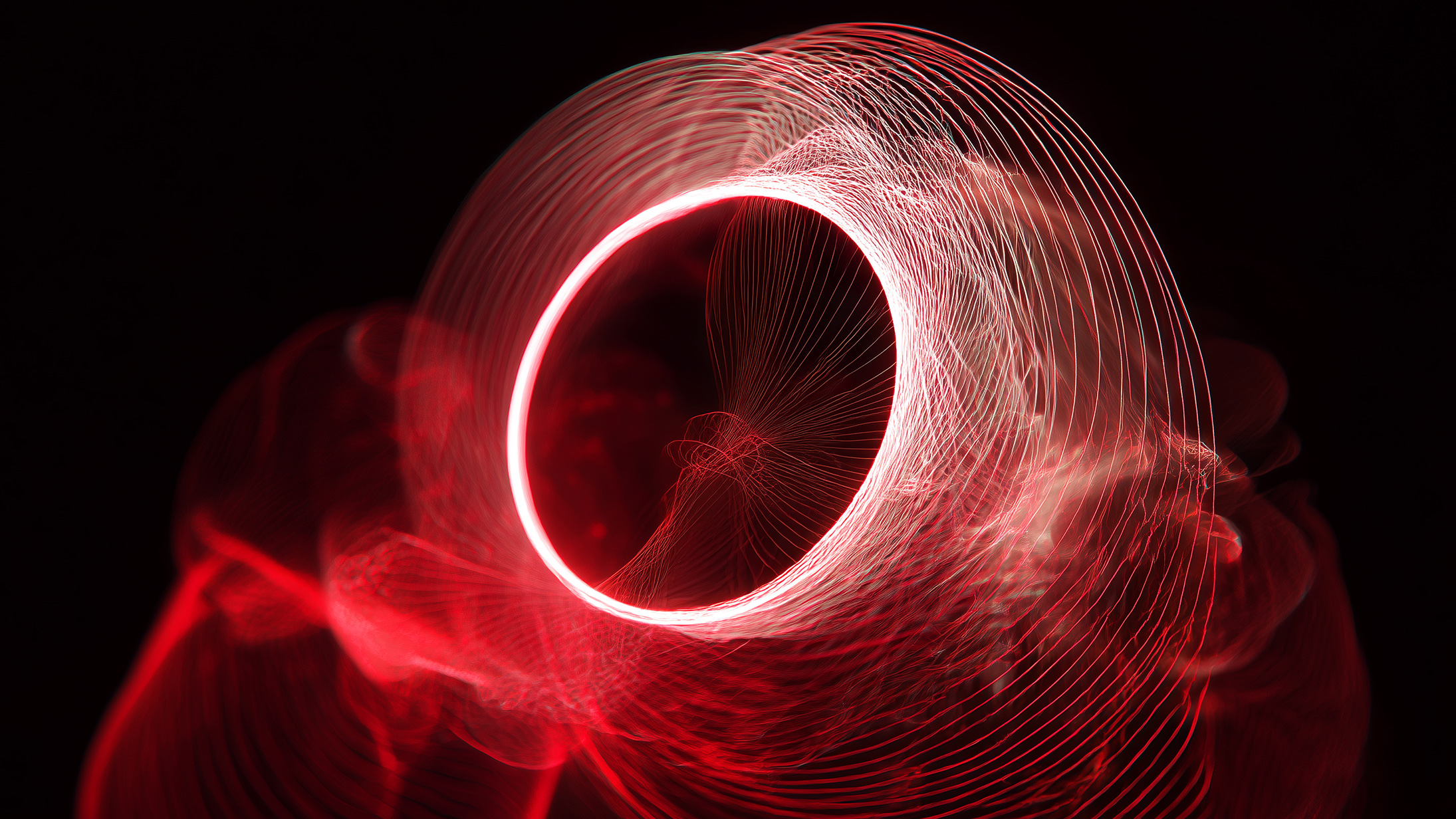
Executive summary:
- AI systems working autonomously: Agentic AI workflows allow multiple AI systems to collaborate, make decisions and execute complex tasks independently in real time
- Natural language comprehension: These systems understand context and intent from conversational language, enabling them to resolve issues like billing disputes immediately rather than requiring customers to wait for human assistance
- Intelligence beyond efficiency: The primary value comes from connecting insights to action, as the AI proactively monitors client behavior, identifies engagement opportunities and creates personalized communications without manual intervention
- Strategic advantage: Organizations implementing these workflows first will gain a substantial competitive edge, operating with a level of speed and intelligence previously unachievable
Think of your business as a brain—processing, adapting and making decisions. But, there’s one problem. In this brain, the neurons are people, each with their own brains, personalities and working styles. And often, manual approvals, endless Slack messages and scattered updates create bottlenecks, leaving you stuck in decision paralysis when execution matters most.
AI agents help by acting as an artificial prefrontal cortex, planning, prioritizing and executing. Unlike self-contained generative AI, which generates ideas, AI agents can also act on them. But without coordination, they remain isolated—almost like a doctor writing a prescription but is walled off from the pharmacy that is supposed to fill it. This is where agentic AI workflows come in. Acting as an enterprise’s nervous system, they connect AI agents, allowing them to work together, adjust in real time and execute autonomously.
Forget the brain metaphor; this concept is real and is something you should be thinking about as you prepare your teams for an AI future.
Here’s how agentic AI workflows will redefine business execution, and what you need to know now.
What is an agentic AI workflow?
In technical terms, an agentic AI workflow is a self-directed, multi-agent system where AI entities collaborate dynamically to perceive context, make decisions and execute complex tasks autonomously, adapting in real-time without requiring human intervention.
In layman’s terms, here's another metaphor to explain how an agentic AI workflow would fix a real-world problem.
Think about a rental car dispute.
Let’s say you return your rental car, having filled the tank before drop-off. As you drive out of the lot, you get a notification from your banking app and see an extra $50 fuel charge.
With traditional automation, you’re stuck in a frustrating loop. A chatbot tells you to call support. A rep needs to verify your claim. The back-and-forth takes hours, and maybe—if you persist—you’ll eventually get a refund.
Generative AI makes this process marginally easier by instantly providing the right contact information through chat. AI agents take it a step further by submitting the support ticket for you.
However, an agentic AI workflow would not only get you in touch with customer service, but refund you automatically.
How? Through multiple AI agents integrated with disparate systems and data:
- Customer relationship management agent – Confirms that you are a loyalty member and should receive special treatment
- Billing agent – Cross-checks your receipt against vehicle data
- Dispute resolution agent – Flags the discrepancy, corrects the charge and notifies you quickly.
This is the core of agentic AI: interconnected agents executing decisions autonomously, based on real-time data, without human bottlenecks.
The technical blueprint: What powers agentic AI workflows?
Agentic AI workflows are more than just automation. They function as multi-agent systems , where individual AI entities operate independently yet collaborate dynamically. But what actual technology is required to make these connections?
Here’s another brain/body metaphor that breaks down the key components: The agents, the integration layer, the data repositories and decision engines and the security and compliance modules.
1. The executive function: Autonomous AI agents
Each agent is an autonomous decision-making entity, specializing in a particular function. These could include:
- Machine learning agents – Predict demand, detect anomalies and optimize pricing
- Natural language processing (NLP) agents – Interpret customer requests and communicate between systems
- Computer vision agents – Process visual data, such as document verification or vehicle inspections
- Reinforcement learning agents – Continuously improve decision-making by learning from past actions
Each agent can take action on your behalf, but they need to be integrated with the right systems to do so.
2. The enterprise nervous system: Integration layer
There are a variety of existing enterprise systems involved in the typical workflow, including:
- Enterprise resource planning (ERP) – Ensuring AI-driven decisions align with financial and inventory data
- Customer relationship management (CRM) – Providing AI with customer history and personalization data
- Supply chain management (SCM) – Allowing real-time coordination of inventory, logistics and demand forecasting
- Identity and security platforms – Ensuring compliance and fraud prevention
3. The memory and reflexes: Data repositories and decision engines Agentic workflows also need a unified data layer to function efficiently:
- Graph databases – Allow AI agents to map relationships between customers, suppliers and transactions
- Event-driven architecture – Agents operate based on triggers rather than waiting for batch updates
- AI-powered knowledge graphs – Provide contextual awareness for better decision-making
4. The immune system: Security and compliance modules
AI agents need security, compliance and ethics hard-coded into their workflow.
- AI ethics guardrails – Prevent bias, enforce transparency and ensure explainability
- Regulatory compliance engines – Automatically adapt workflows to legal and policy changes
- Zero trust security layers – Authenticate AI actions and prevent unauthorized data access
Learn about Publicis Sapient’s agentic AI workflow for software development, Sapient Slingshot
Agentic AI workflows example: The proactive salesperson
While no agentic AI workflow is easy to implement, the most frequently discussed “low-hanging fruit” for agentic AI workflows is customer service. We can all unanimously agree that customer support is broken for customers, employees and companies. If businesses can successfully scale agentic AI at the right cost and with the right balance of human oversight, it will shift from an expense to a strategic advantage and even a growth driver.
But what about the workflows that aren’t so obvious to the customer, the ones that are internal? These behind-the-scenes workflows are not only ripe for disruption, but also don’t rely on consumer trust in AI overall.
Think about the B2B sales process—overburdened sales reps juggle hundreds of cold calls every day, frantically looking for their customer data across five different systems, trying to keep messy CRM systems up to date and trying to remember to check in on their endless digital rolodex of current clients.
Here’s where the agentic AI workflow comes in:
An overview
- The research agent: Automatically searches for and collects relevant business information across target client press releases, news articles, financial reports and internal product updates
- The CRM agent: Monitors top target client activity, from client emails, to meetings, to email opens and more
- The relationship agent: Alerts you when business information is relevant to a top target client, citing how they might be interested in a new service offering or an industry trend
- The outreach agent: Drafts an email to your top target client based on the business opportunity and invites your client to schedule a conversation
Data flow
This system enables end-to-end automation by:
- Collecting external business data
- Monitoring client activity across channels
- Identifying new business opportunities
- Drafting personalized outreach
Each step builds on the last to help you act on the right opportunities, faster.
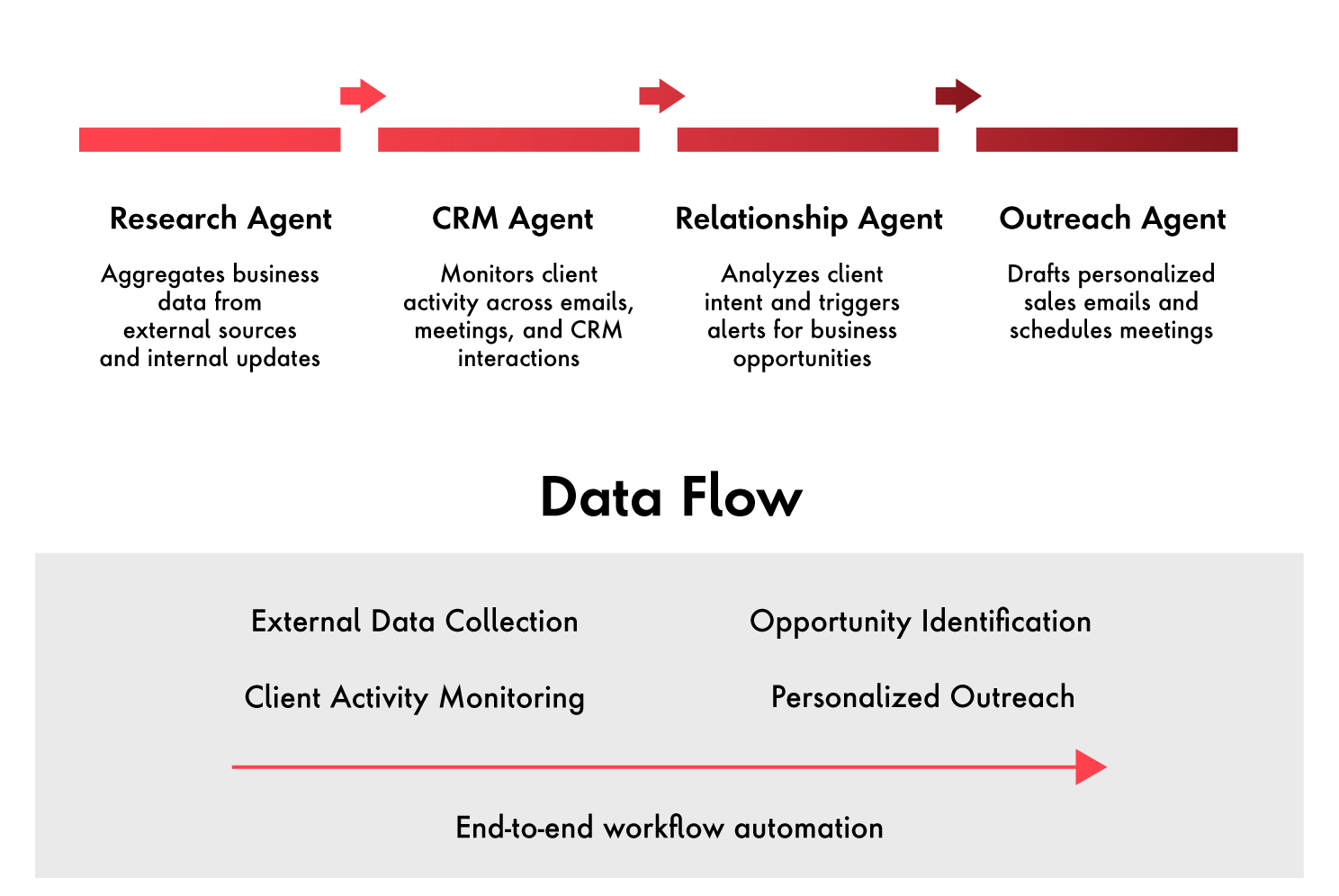
The agent breakdown
Agent 1: The research agent
Function:
Aggregates relevant business data from external sources (news, financial reports, press releases) and internal updates (product changes, new offerings).
Key AI components:
- Web scraping & data aggregation: APIs to extract business information
- Natural language processing (NLP): Transformers for entity recognition and summarization
- Knowledge graphs: Serverless graph database for mapping company relationships and historical engagements
Integration flow:
- Scheduled job triggers API calls to financial data sources and news aggregators
- NLP models classify and extract relevant insights (e.g., acquisitions, executive moves, funding rounds)
- Data is stored in a vector database for retrieval
- Event-driven architecture updates relevant client profiles in the CRM
Agent 2: The CRM agent
Function:
Monitors target client activity, tracking engagement signals across emails, meetings and CRM interactions.
Key AI components:
- Behavioral data analysis: Uses anomaly detection via ML models
- Real-time event processing: Open-source data processing platform for tracking user interactions
- Email parsing & engagement tracking: API for email metadata analysis
Integration flow:
- Webhook listeners capture CRM events (e.g., emails opened, meetings scheduled)
- ML-based sentiment analysis models score client intent
- Signals trigger alerts for the relationship agent
Agent 3: The relationship agent
Function:
Analyzes client intent and triggers alerts when an opportunity arises.
Key AI components:
- Predictive analytics: LSTMs or time-series forecasting models (Prophet, AWS Forecast) analyze engagement trends
- Contextual AI: LLMs (GPT-4, Claude) link external news with CRM activity
- Graph database for client relationship modeling: Neo4j maps historical interactions and inferred client needs
Integration flow:
- CRM agent feeds engagement signals
- Research agent provides external news context
- AI generates a personalized business insight for the sales rep
Agent 4: The outreach agent
Function:
Autonomously drafts personalized sales outreach emails and schedules meetings.
Key AI Components:
- Generative AI (LLMs): GPT-4, Cohere for email personalization
- Intent detection models: Classify email tone and sentiment
- Scheduling optimization: Reinforcement Learning (RL) models adjust timing for higher response rates
Integration Flow:
- Relationship agent provides contextual insights
- LLM generates a tailored sales email based on engagement patterns
- AI agent interfaces with calendar APIs to propose meeting slots
The functionality breakdown
Aside from the particular agents required for this sales workflow, there are also four key functionalities that this agentic AI workflow requires: enterprise integrations, infrastructure considerations, AI orchestration and data privacy and compliance.
Core enterprise integrations:
These integrations provide comprehensive real-time data access, enable automated workflows across systems, and maximize ROI by extending AI capabilities throughout existing enterprise infrastructure.
- CRM platforms: Salesforce, HubSpot, Microsoft Dynamics (primary data source for client interactions)
- Marketing automation tools: Marketo, Pardot, Eloqua (to track email engagement and lead scoring)
- Enterprise communication systems: Outlook, Gmail, Slack (for monitoring client interactions)
- Financial data sources: Bloomberg, Reuters, AlphaSense (for pulling market insights)
- News and industry reports: APIs like Google News, LexisNexis and company press release feeds
- Scheduling and calendaring: Google Calendar, Microsoft Exchange (for AI-driven meeting scheduling)
Infrastructure considerations
These are the technical foundation and architecture required for deploying agentic AI systems, consisting of cloud-native environments on major providers (AWS, Azure, GCP) with serverless functions, event-driven integration for real-time data streaming, centralized data warehousing and graph databases for relationship mapping.
- Cloud-native architecture: Agents should run on major cloud service providers with serverless function platforms for scalability
- Event-driven integration: Use message brokers and event streaming platforms for real-time data streaming
- Data warehousing: Centralized data processing in cloud-based data warehouse solutions
- Graph database for relationship mapping: Specialized databases designed to store and visualize complex relationships for tracking client-company interactions
AI orchestration and execution
This is the coordination and deployment systems for managing multiple AI agents, utilizing specialized frameworks for workflow orchestration alongside containerization technologies for scalable deployment. This approach enables efficient management of complex multi-agent workflows and ensures reliable, scalable performance across varying workloads.
- Agent orchestration frameworks: Applications like Ray, FastAPI or Temporal.io to coordinate agent workflow
- Containerization: Agents deployed in Kubernetes clusters for scalability
Data privacy and compliance
The regulatory frameworks and technical controls that govern how AI agents access, process and store sensitive information, including PII anonymization protocols, robust access control mechanisms and comprehensive audit logging systems. These measures ensure AI operations maintain legal compliance, protect user privacy and provide transparent accountability for all automated actions across the enterprise.
- General Data Protection Regulation (GDPR) and California Consumer Privacy Act (CCPA) compliance: Implement Personally Identifiable Information (PII) anonymization techniques to protect sensitive user data and meet legal requirements
- Access control: Implement authentication protocols and Identity and Access Management (IAM) role-based permissions to prevent unauthorized agent actions and ensure appropriate data access levels
- Audit logging: Record all AI-driven actions in Security Information and Event Management (SIEM) solutions to maintain comprehensive activity trails for security monitoring and compliance verification
Other applications of agentic AI workflows
Similar to how B2B sales progresses through defined stages of prospecting, qualifying, demonstrating value and closing deals, this workflow approach can be applied to many other functional processes, including:
- Business analysts can structure insight collection and recommendation development
- Marketing managers can systematize content creation and distribution
- Financial analysts can approach forecast modeling and variance analysis
- IT support specialists can manage ticket resolution processes
- Developers can organize feature development and testing cycles
Each leverages the same principles of relationship development, stakeholder management and stage-based progression toward a defined outcome.
Maturity checklist: Are you ready for agentic AI workflows?
Clearly, agentic AI workflows are complex to implement but deliver quantifiable value: reducing manual processing time by 70-85 percent, operating 24/7 with over 90 percent accuracy, and effectively handling work that would require three to five full-time employees (according to internal Publicis Sapient research).
This automation ultimately frees sales professionals to focus on what humans do best: building relationships, applying strategic creativity and delivering the emotional intelligence that drives true customer success.
But before taking a deep dive into the mechanics of the workflow, technical leaders should ask themselves a few questions to evaluate how “ready” their organizations, including people, technology and processes, are to invest in an agentic ai workflow.
1. Interoperability
- Does your existing tech stack have any legacy systems that inhibit integration efforts?
- Are APIs and event-driven architectures in place?
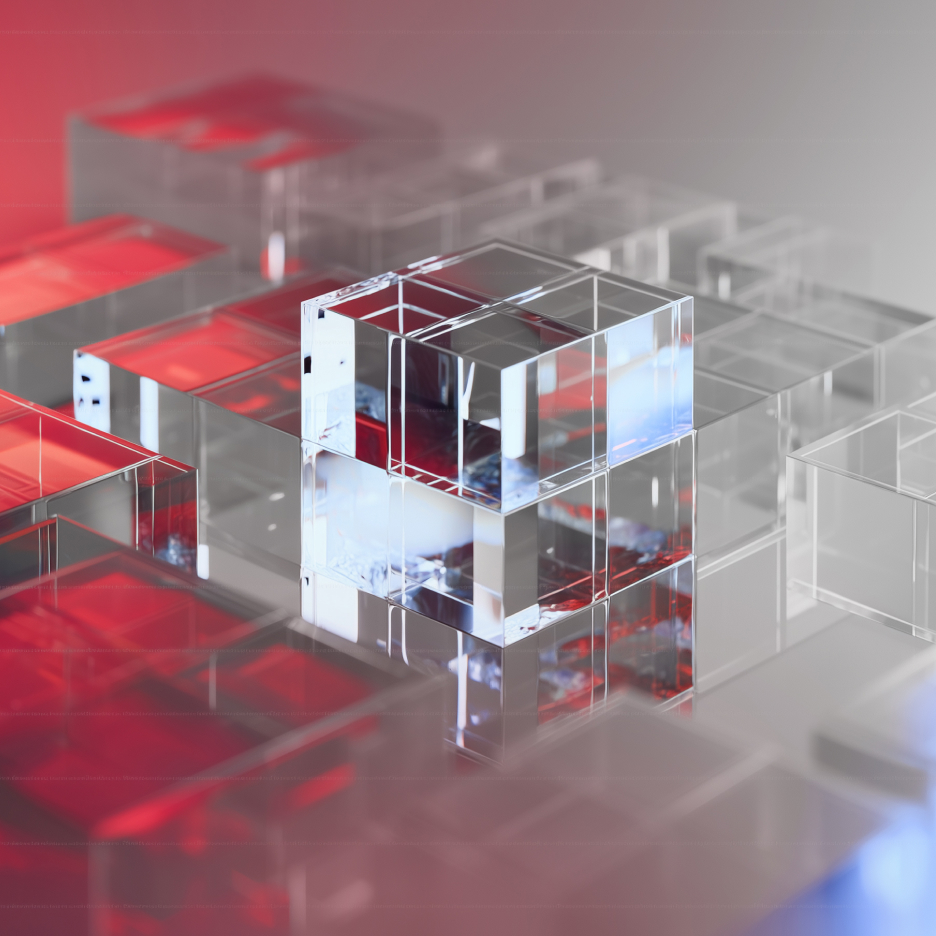
2. Scalability
- Are you able to identify the pain points in your current workflow that could best be eased with AI agents?
- Are your cloud and data infrastructures optimized for AI-native operations?
3. Human oversight and trust
- What level of human-in-the-loop governance is required?
- How do you maintain visibility into agentic decisions?
4. Security and ethical considerations
- How are AI-driven actions logged, audited and secured?
- Are risk management policies in place for AI-generated errors and accountability?
“If you come up with an idea for an AI agent and begin building it without any plan for integration, you’re going to face vast infrastructure hurdles, and might just end up right back where you started.” -Andy Maskin, Director, AI Creative Technology, Publicis Sapient
Getting started: Your agentic AI workflow roadmap
Even if your answer to every question above is clear and confident, the truth is: Most companies will need outside experts in order to begin their agentic AI journey. While the future of agentic AI promises millions of dollars saved, higher customer satisfaction scores, new business and more, the journey is more efficient when you have experts on your side.
Here’s a rough outline of what an implementation roadmap would look like, using the example of our “proactive salesperson” agentic AI workflow.
Discovery and technical assessment
Before implementation, a deep evaluation of the current IT infrastructure is necessary to identify integration points and security risks. This includes:
- Auditing core enterprise systems like CRM platforms such as Salesforce or Microsoft Dynamics, ERP systems like SAP or Oracle, and communication tools such as Outlook or Microsoft Teams
- Mapping out data flow to ensure AI agents can access structured and unstructured data in real time
- Assessing AI readiness to determine whether existing infrastructure supports event-driven workflows or if cloud modernization is required
- Reviewing security and compliance to ensure AI-driven processes align with GDPR, CCPA and internal governance policies
By the end of this phase, enterprises will have a technical blueprint outlining the integration strategy and AI workflow design.
Proof of concept
Instead of immediately deploying a full-scale AI system, a proof of concept (PoC) should be tested in a controlled environment with limited users and data sets.
In this phase, two key AI agents can be introduced:
- The research agent—Uses natural language processing and web scraping to gather relevant business intelligence from press releases, financial reports and internal product updates
- The CRM agent—Tracks client interactions across email, meetings and CRM updates using AI-driven behavioral analytics
To support these agents, the technical team must:
- Deploy an event-driven architecture for real-time CRM data streaming
- Implement a graph database to track client relationships over time
- Set up an observability layer for AI logging and debugging
The success of this phase depends on validating whether AI-driven research and CRM insights can meaningfully augment human decision-making in sales workflows.
Expanding the prototype into full execution
Once the PoC is validated, the AI workflow can be expanded to automate full sales execution.
This phase introduces two more AI agents:
- The relationship agent—Analyzes CRM and research data to predict client needs and alert sales representatives to engagement opportunities
- The outreach agent —Uses large language models such as GPT-4 or Claude to generate personalized sales emails and suggest meeting times
To make these agents operational at scale, CIOs must:
- Enable real-time data synchronization across CRM and ERP systems
- Implement AI governance mechanisms to introduce human oversight at key decision points
- Apply security protocols such as zero-trust access controls and bias detection models to ensure AI-generated content aligns with regulatory requirements
At this stage, the enterprise will have a fully operational AI-powered sales workflow that minimizes manual tasks and accelerates deal cycles.
Continuous optimization and scaling
Even after deployment, AI workflows require continuous optimization and scaling.
Ongoing efforts should include:
- Reimplementing LLMs to improve response accuracy and personalization
- AI drift monitoring to ensure models stay aligned with business objectives
- Performance tracking to measure the impact of AI on lead conversion rates, sales cycle efficiency and cost savings
The full potential of the artificial brain
The human brain is built for efficiency. It does not waste energy on redundant processes or slow down because of bottlenecks. It adapts, learns and automates where necessary, keeping everything running without conscious effort.
In the same way, businesses can evolve beyond fragmented, manual processes and create systems that move instinctively. The future of agentic AI workflows is not just about efficiency but about freeing leaders and employees from the burden of operational complexity, allowing us to execute at the speed of their intelligence.
For CEOs, these workflows will surface the most critical insights and decisions, cutting through the noise so they can focus on strategy and innovation.
CIOs will no longer be consumed by infrastructure management, as AI dynamically scales systems and corrects inefficiencies in real time.
Sales leaders will know exactly when to engage a client before competitors do, while finance teams will anticipate market shifts before they happen.
Employees will spend less time navigating administrative bottlenecks and more time on creative, high-impact work that drives real value.
These workflows will not only accelerate business performance but will also empower us to focus on what truly matters, shaping a future where work is smarter, decisions are clearer and our human potential is fully realized.
Related Reading
-
-
Sapient AI Solutions
Transform your business with tailored AI solutions designed to unlock unique value, drive innovation and improve efficiency.
-
The Top 5 Generative AI Trends in 2025
How to revolutionize business with AI agents, content supply chains and workforce transformation.